All our events
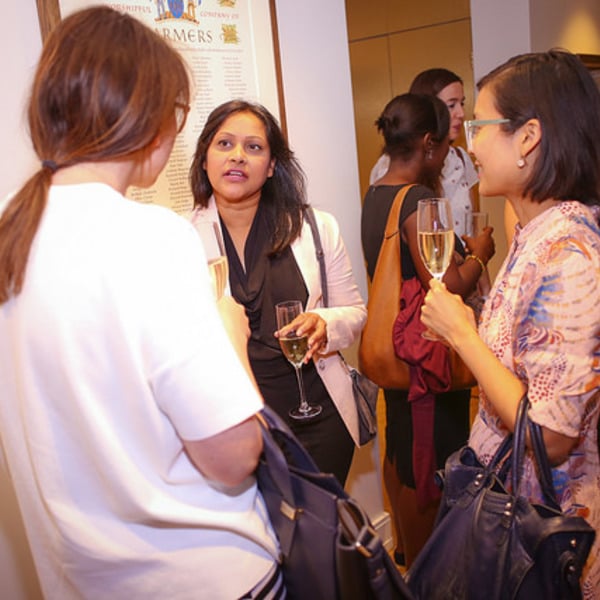
Women in AI & Data Reception
Women in AI & Data Reception
Inspiring and influential women in AI will come together for an evening of networking and presentations. Join us for a night of great food & wine, and contribute to supporting women in the field. The reception is open to all genders.
London
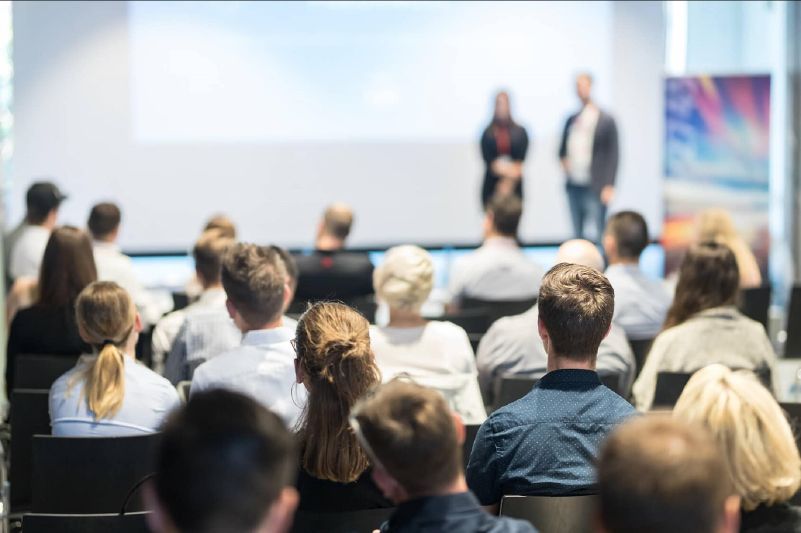
Part of the Berlin AI Summit
Deep Learning Summit
Explore how deep learning will impact and solve challenges in industry and society through diverse applications.
Berlin
04 - 05 October 2022
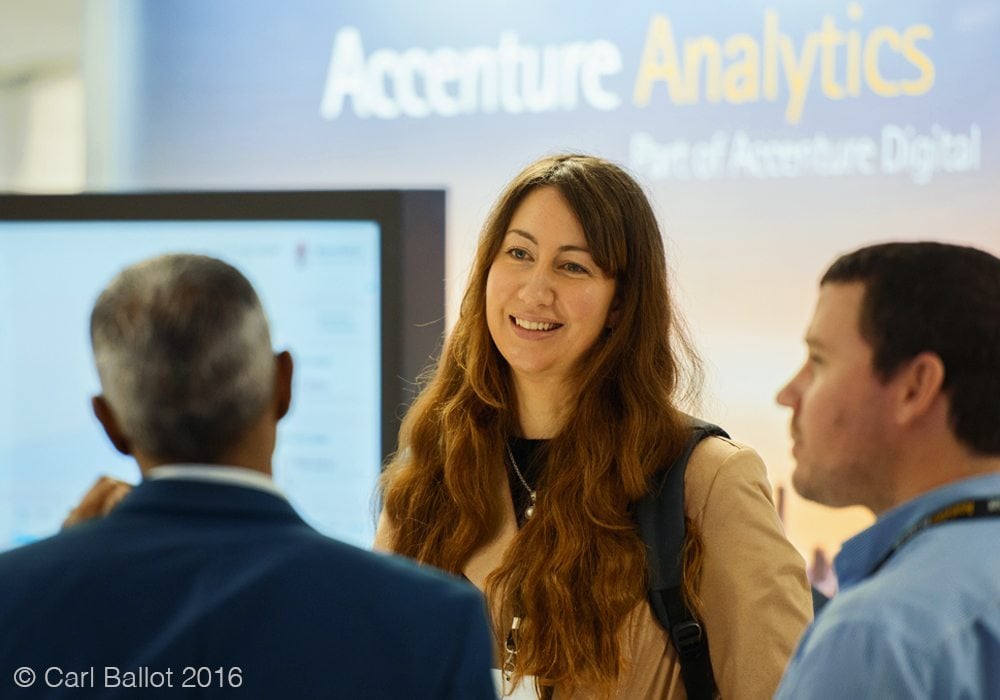
Part of the Berlin AI Summit
Enterprise AI Summit
The Enterprise AI Summit brings together senior-level business executives and data practitioners to explore AI applications in the real-world to transform your ROI, increase efficiencies and ensure scalability.
Berlin
04 - 05 October 2022
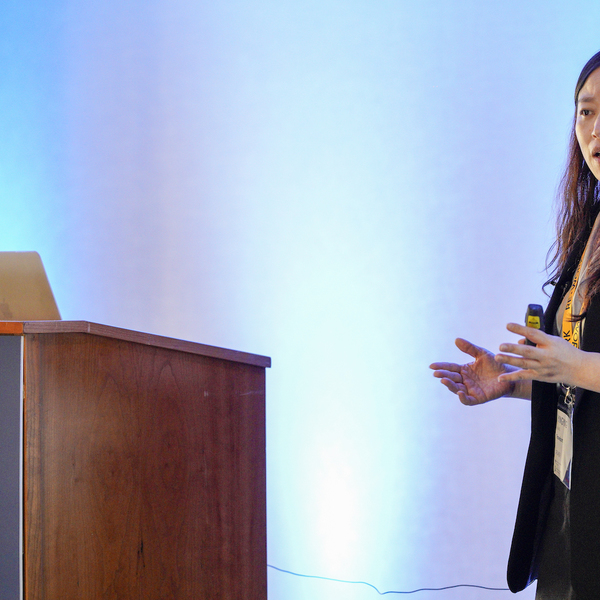
MLOps Summit
The MLOps Summit will explore how to build an effective MLOps infrastructure and take advantage of innovative solutions for data lifecycle management. What are the best practices for efficiently training & deploying models? How can DevOps methodology be successfully applied in the ML workflow?
London
09 - 10 November 2022
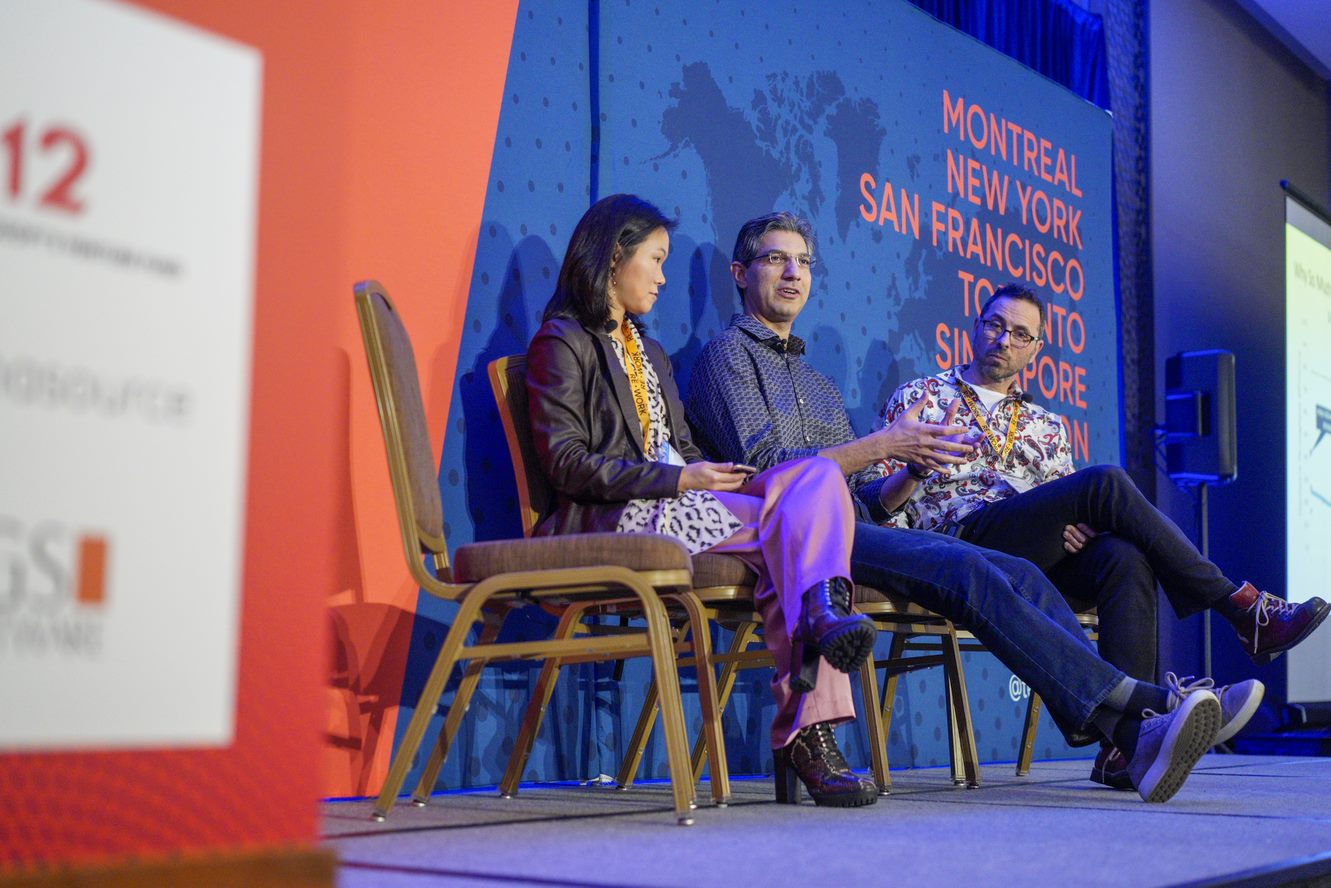
Conversational AI Summit
This summit will bring together experts in the field of conversational AI to discuss the latest advancements, challenges, and best practices in designing, developing, and deploying conversational AI systems. The event will feature keynote speakers, workshops, and panel discussions covering topics such as natural language processing, chatbot development, voice assistants, and the ethical considerations of conversational AI.
London
16-17 May 2023
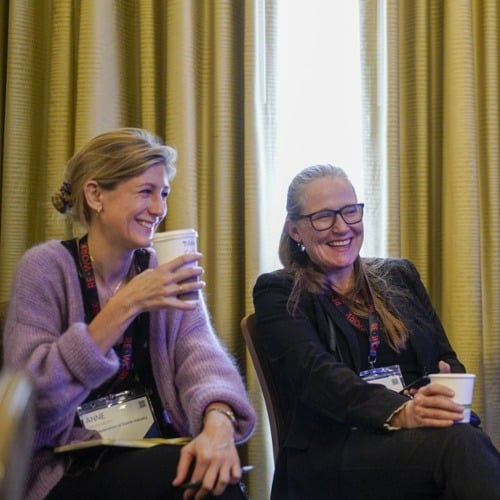
AI in Finance Summit London
Explore the latest AI tools & techniques and their application in the financial sector with data scientists, ML engineers, researchers & CTOs across the industry. Applications include wealth and portfolio management, retail banking, trading & payments innovation.
London
22 - 23 May 2024
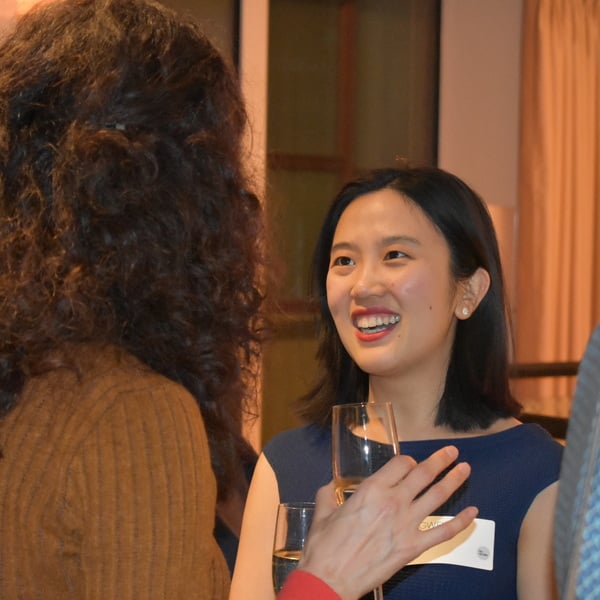
Women In AI & Data Reception
Join us for an evening of thought-provoking discussions, presentations and a networking reception. Support the achievements and advancement of women within the AI industry. The evening is open to all genders!
London
24 January, 2023
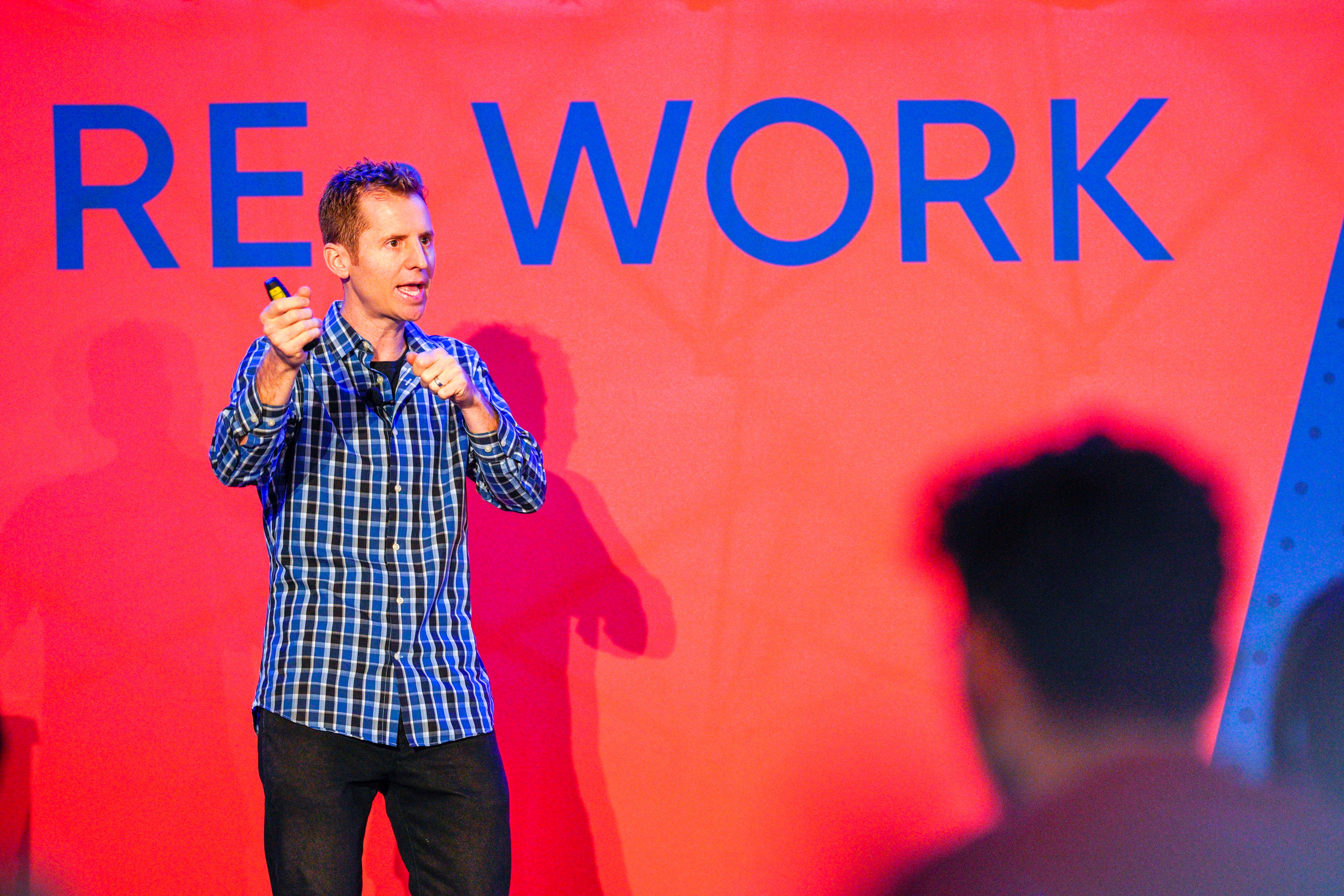
Deep Learning Summit London
This summit is a two-day event that will bring together experts and enthusiasts in the field of Deep Learning to discuss the latest trends, techniques, and applications of this cutting-edge technology. The event will feature keynote speeches, panel discussions, and technical sessions on a range of topics, including neural networks, reinforcement learning, natural language processing, computer vision, and more.
London
4-5 November 2024
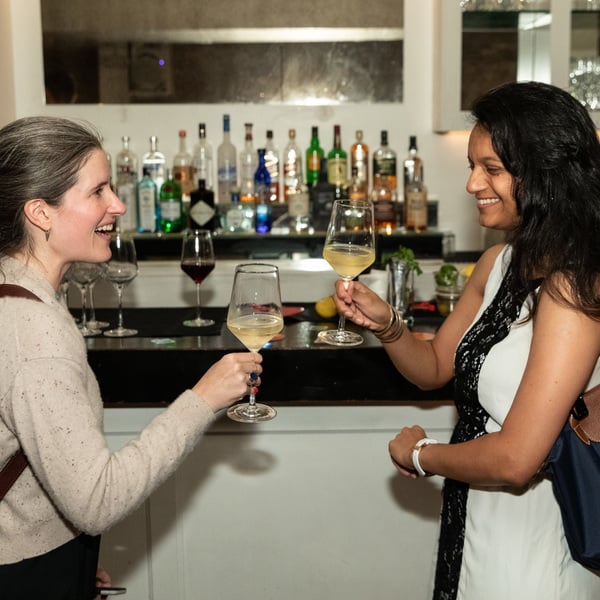
Women in AI Reception
Leading minds in artificial intelligence will come together for an evening of networking and keynote presentations. Join us for a three course dinner to support women in AI and Machine Intelligence.
Toronto
9 November 2022
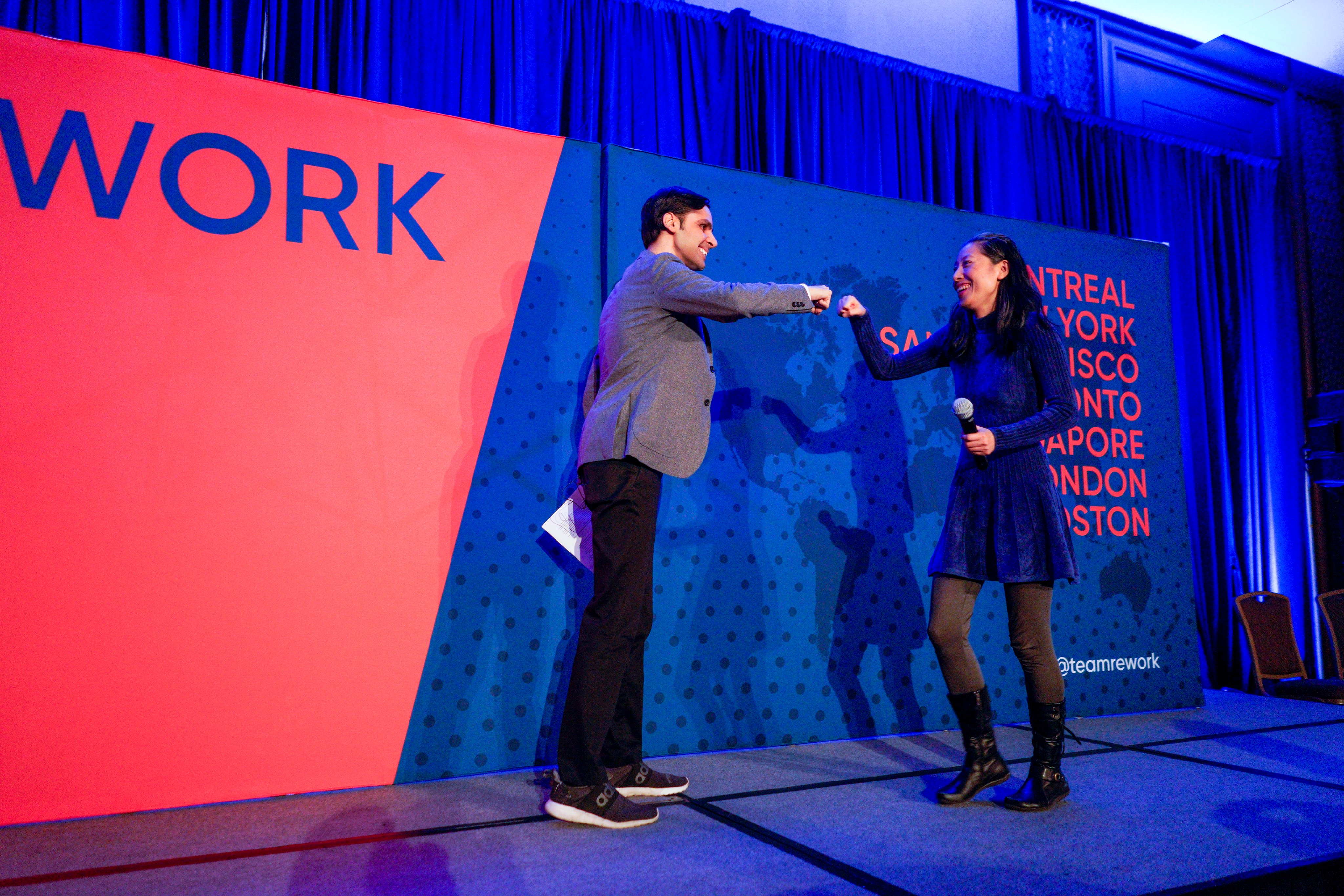
AI in Finance Summit New York
Explore the latest AI tools & techniques and their applications with top speakers from the banking, insurance, academia and the financial sector. CTOs, Directors, ML engineers, Data Scientists and Researchers will share their insights into recent breakthroughs in technical advancements and fintech applications across the industry.
New York
April 18-19, 2024
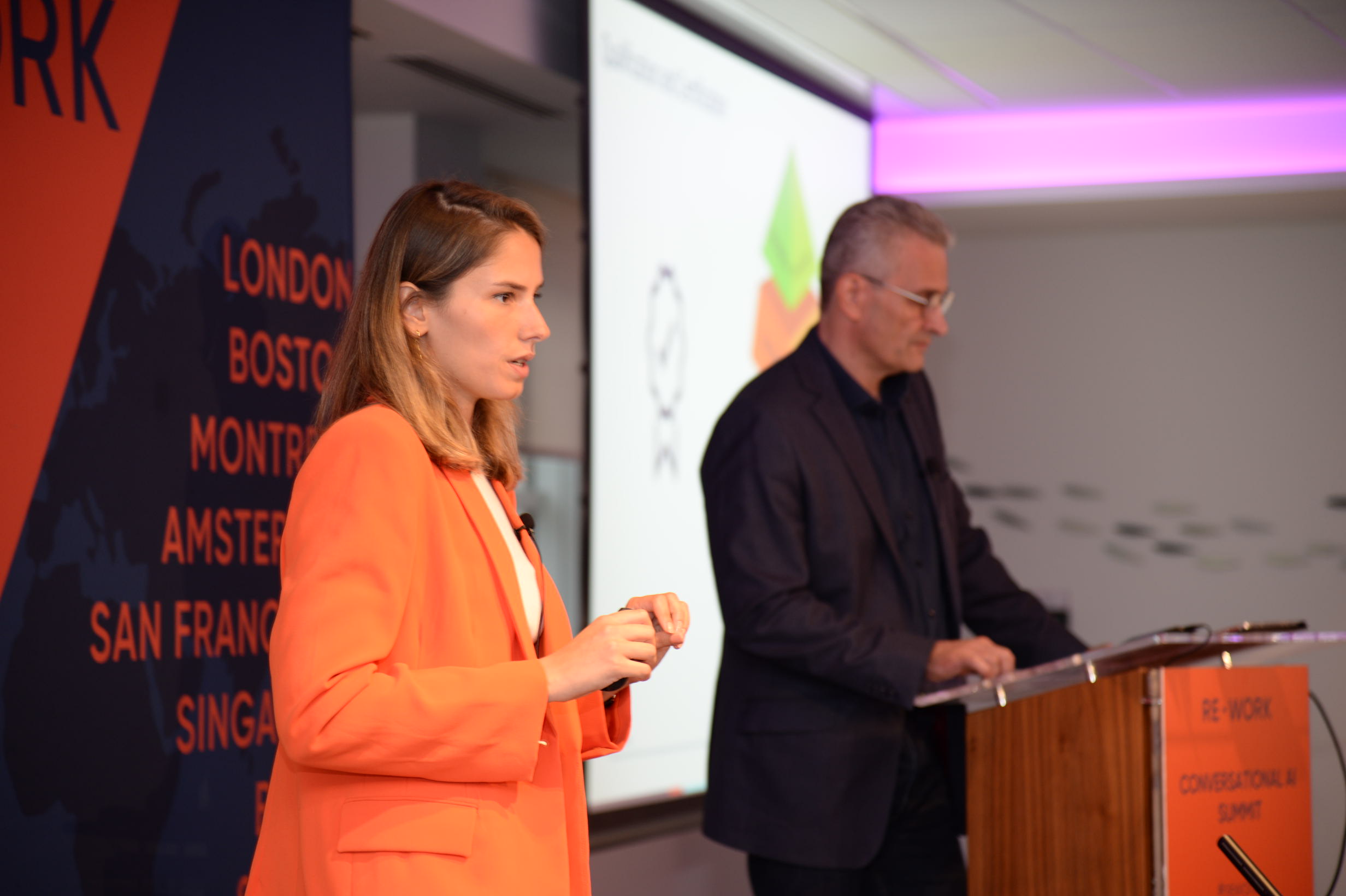
AI Summit West
AI Summit West explores the latest breakthroughs from researchers in the field of Deep Learning. Discover ways to overcome obstacles in developing and deploying deep neural networks as well as novel algorithmic advancements from leading academics and industry professionals.
Santa Clara
February 13-14, 2024
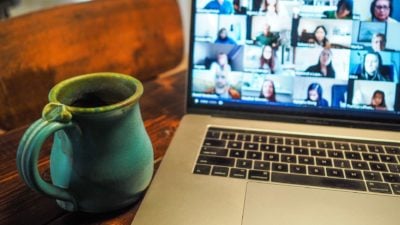
Virtual AI Summit
An interactive, online experience with thought-provoking discussions and insights from global thought leaders bringing you the latest developments, prospects and innovations across Artificial Intelligence & Machine Learning
Virtual / Online
January 30-31, 2023
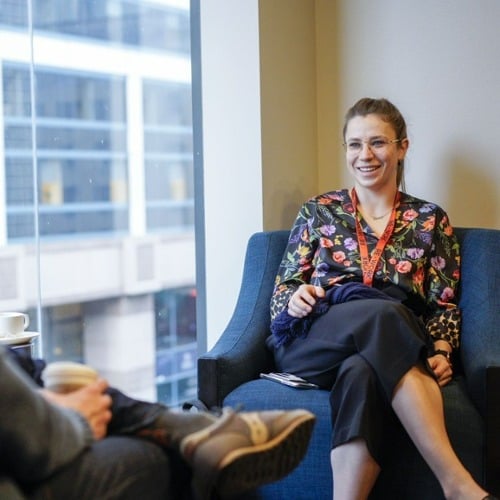
Generative AI Summit London
Discover the limitless possibilities of generative AI applications. From creating stunning artworks to generating personalized music compositions, the potential of generative AI knows no bounds. Unleash your creativity and dive into the world of AI-driven innovation. Let us guide you through the captivating realm of generative AI applications and unlock the future possibilities that await.
London
May 22 - 23 2024
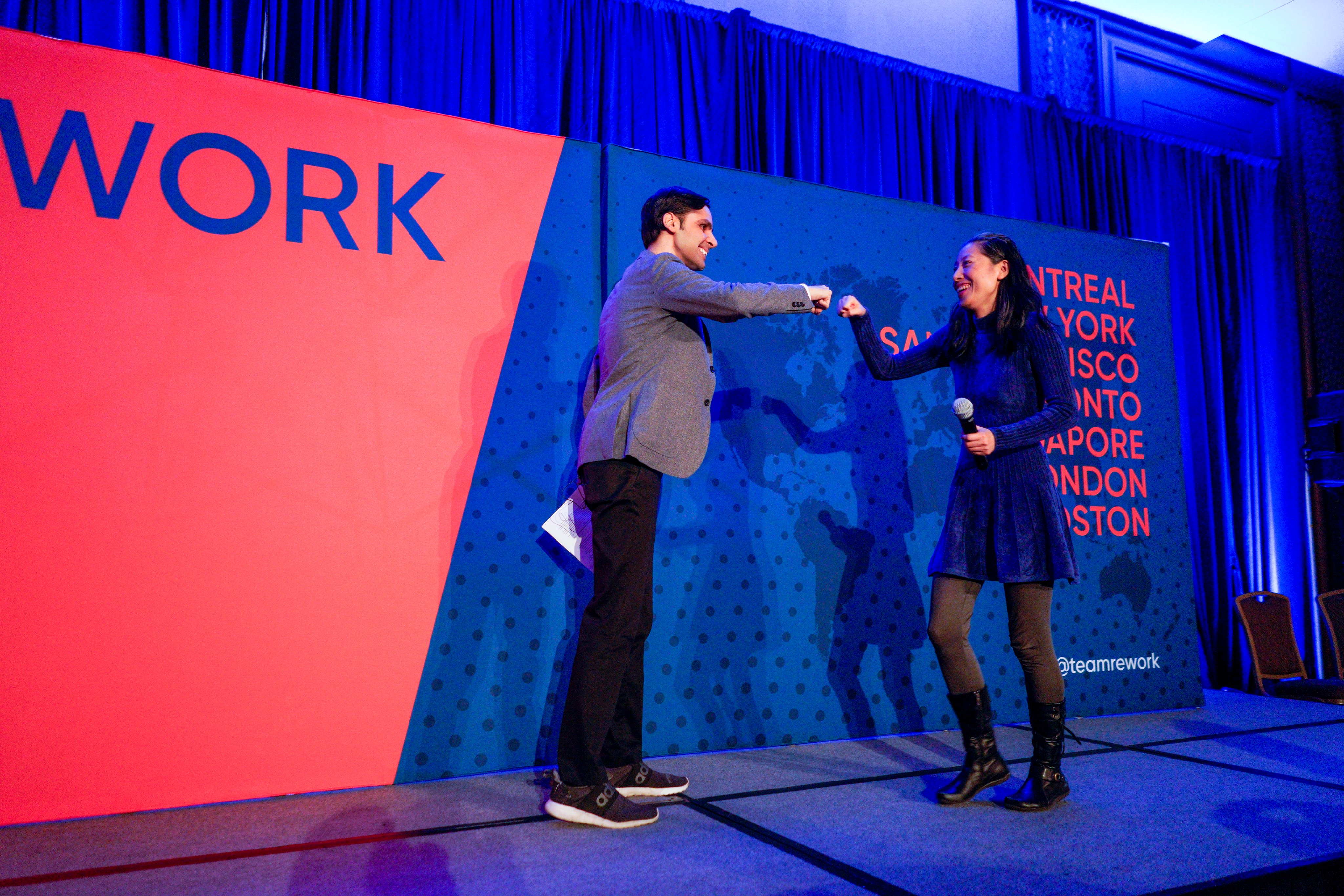
AI in Healthcare & Pharma Summit
This summit will showcase the latest advancements in AI technologies and their applications in healthcare. Speakers will discuss the potential of AI to improve patient outcomes, streamline clinical workflows, and address healthcare challenges such as the COVID-19 pandemic. The conference will also address the ethical considerations of AI in healthcare, such as data privacy and bias, and how to ensure that AI technologies are developed and deployed in a responsible manner.
Boston
November 13-14, 2024
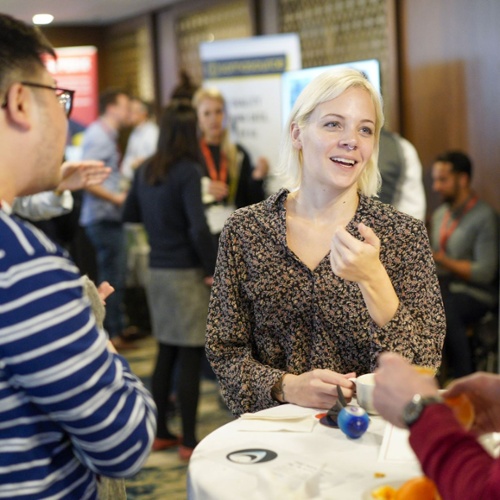
Nordics AI Summit
Explore the latest breakthroughs from researchers in the fields of Deep Learning and Enterprise AI. Discover ways to overcome obstacles in developing and deploying deep neural networks as well as novel algorithmic advancements from leading academics and industry professionals.
Stockholm
November 29-30, 2022
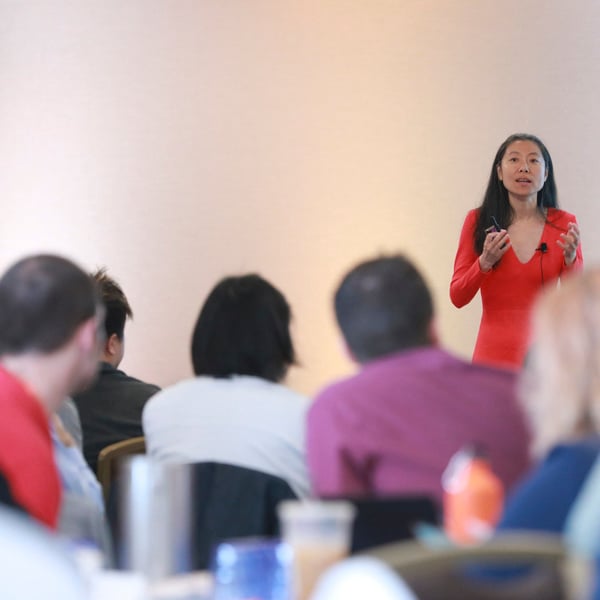
Part of the Toronto AI Summit
AI in Finance Summit
The AI in Finance Summit brings together data scientists, engineers, CTOs, CEOs & leading financial corporations to explore the impact of AI in the financial sector. Applications include identifying and preventing risks, revolutionising financial forecasting & compliance.
Toronto
November, 09 - 10, 2022
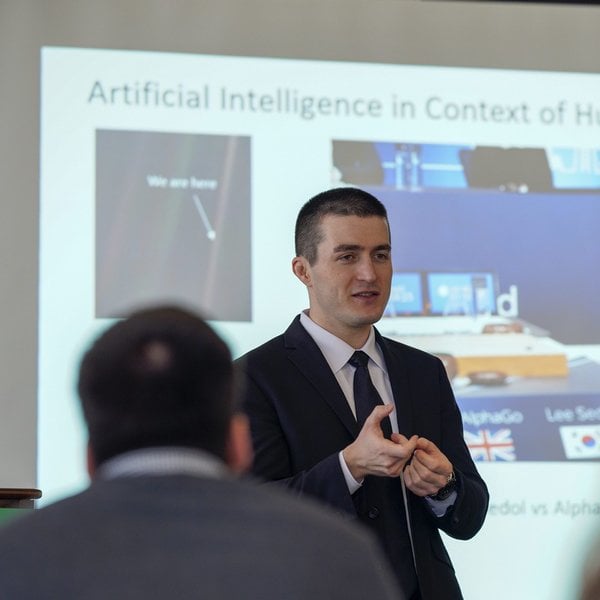
Part of the Toronto AI Summit
Deep Learning Summit
The Deep Learning Summit is the next revolution in AI. The increasingly popular branch of machine learning explores advances in methods such as image analysis, GANs, NLP, and neural network research. This summit will explore the latest research advancements and trends from global pioneers in the ...
Toronto
November, 09 - 10, 2022
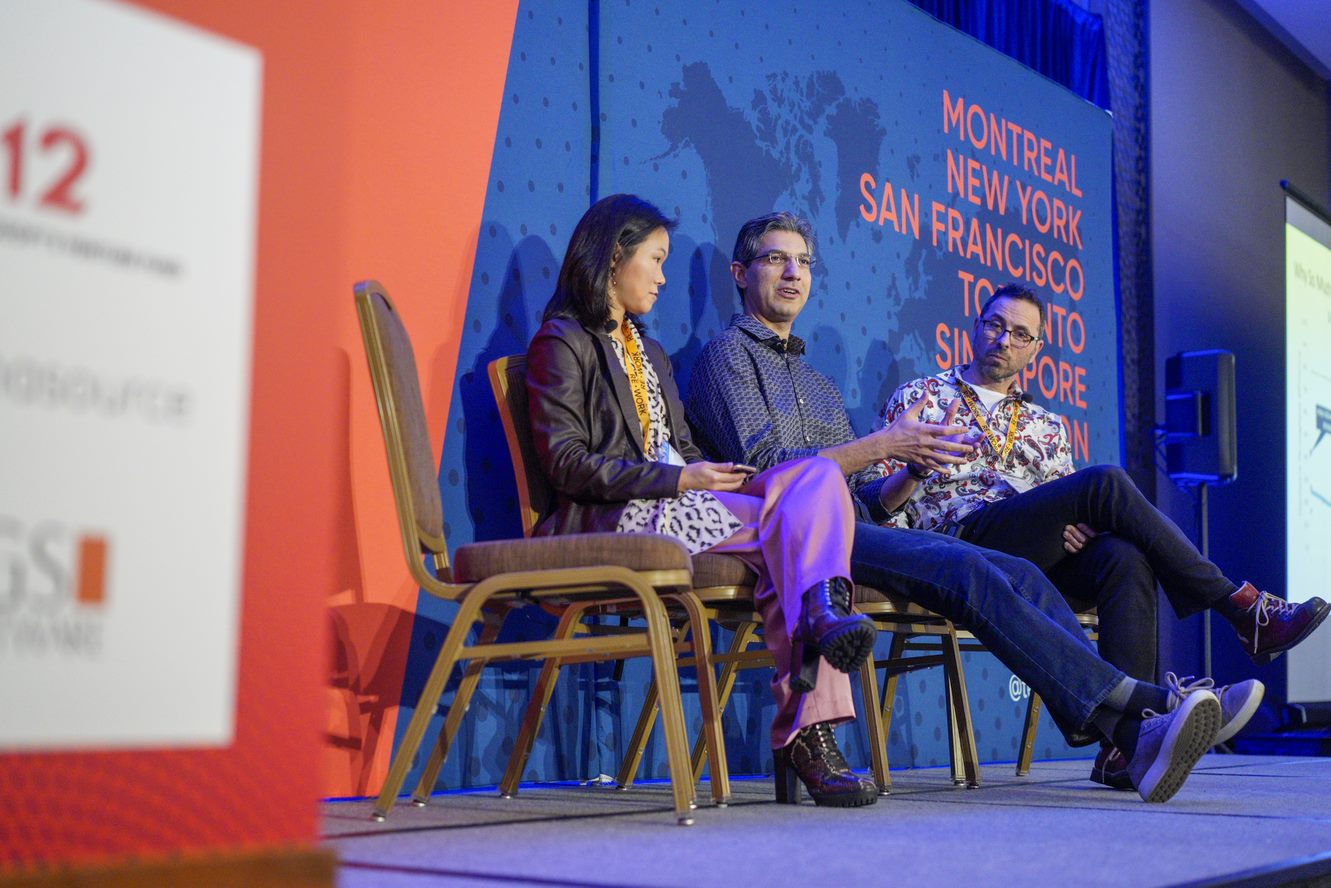
AI in Finance Summit Toronto
Explore the latest AI tools & techniques and their applications with top speakers from the banking, insurance, academia and the financial sector. CTOs, Directors, ML engineers, Data Scientists and Researchers will share their insights into recent breakthroughs in technical advancements and fintech applications across the industry.
Toronto
October 23-24, 2024
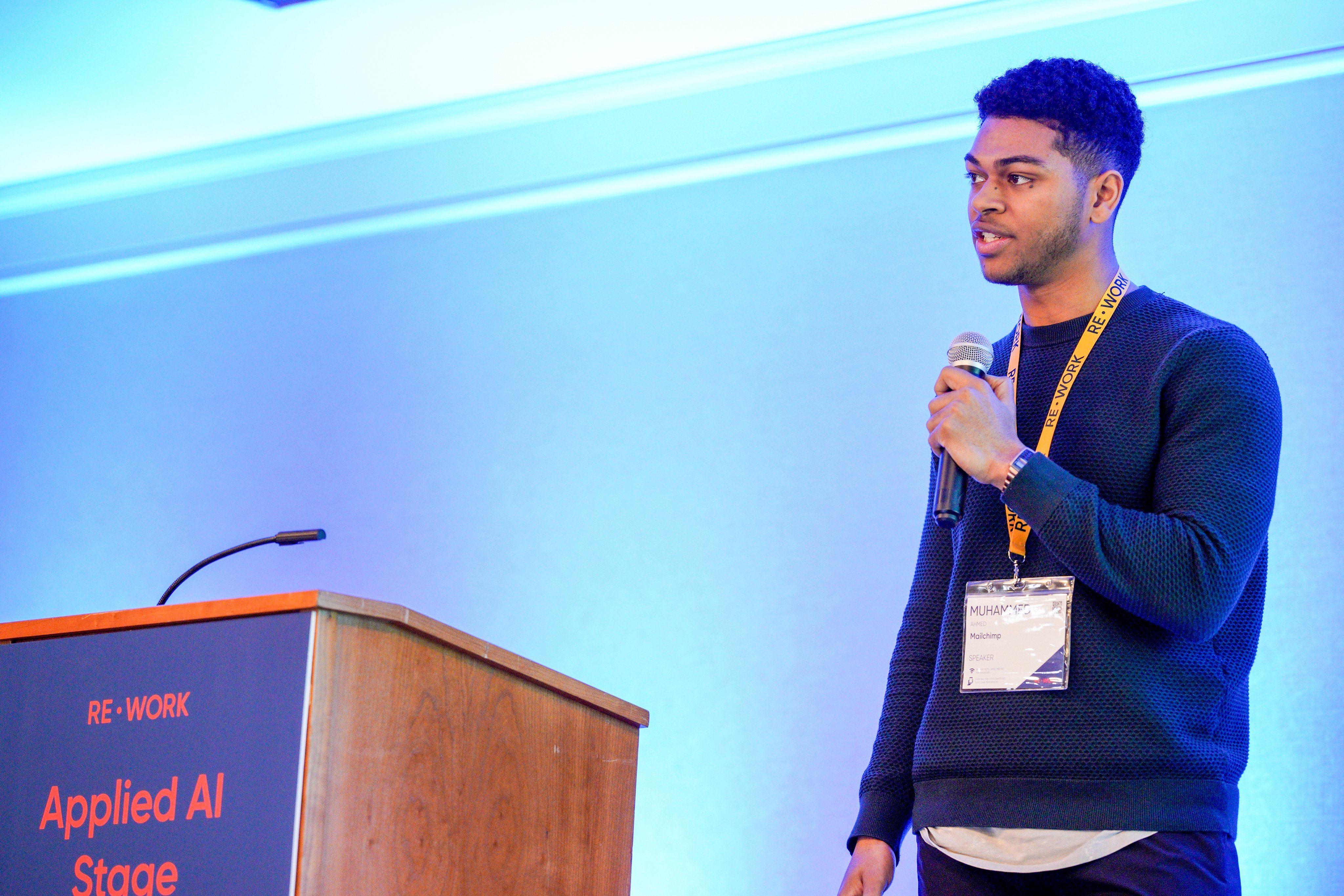
Deep Learning Summit Toronto
The Deep Learning Summit is the next revolution in artificial intelligence. The increasingly popular branch of machine learning explores advances in methods such as image analysis, speech and pattern recognition, natural language processing, and neural network research. This summit will explore t...
Toronto
October 23-24, 2024
.jpg)
Data Architecture Summit
Discover the limitless possibilities of generative AI applications. From creating stunning artworks to generating personalized music compositions, the potential of generative AI knows no bounds. Unleash your creativity and dive into the world of AI-driven innovation. Let us guide you through the captivating realm of generative AI applications and unlock the future possibilities that await.
London
September 11 2024
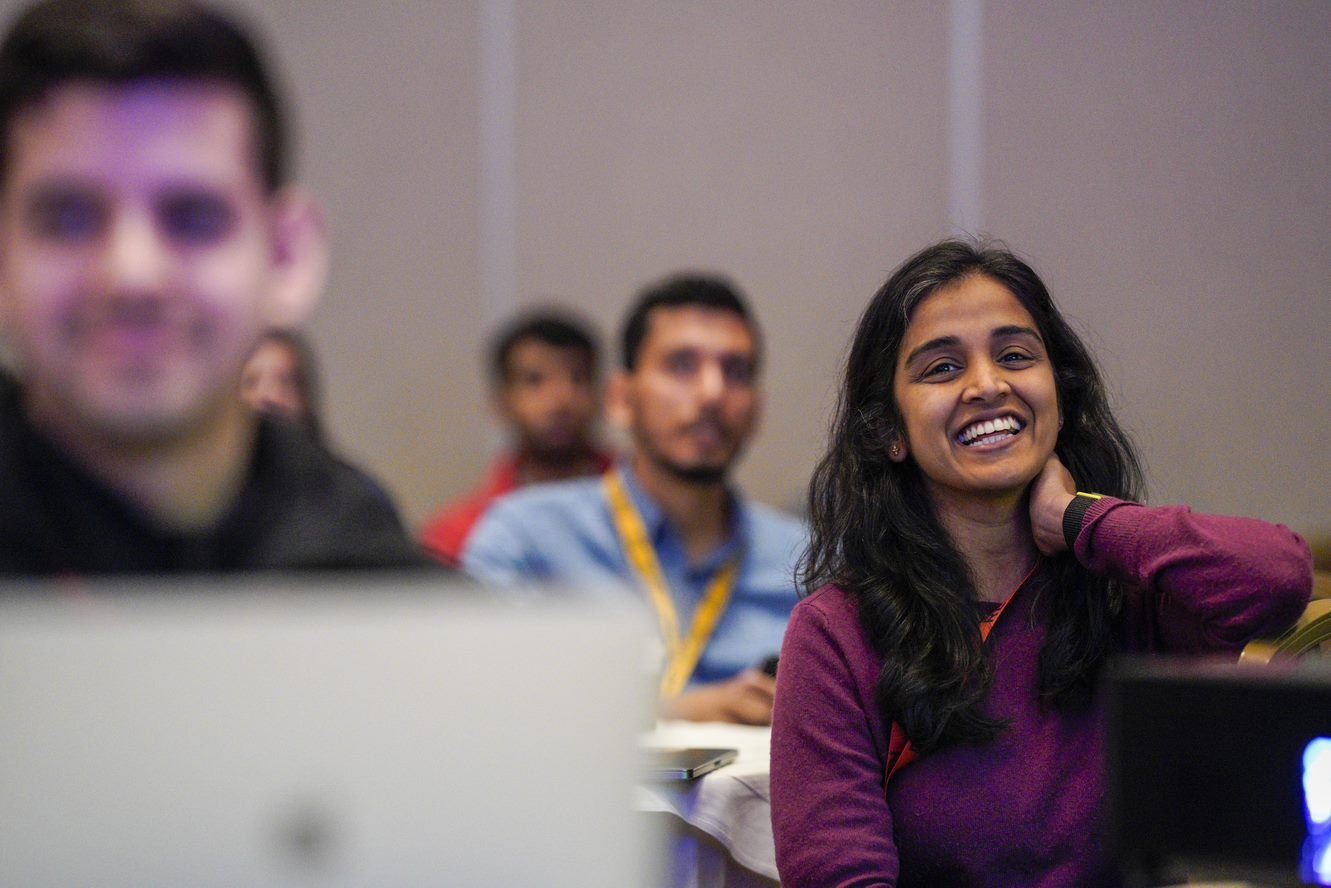
CDAO FS London
Join the UK's most senior event for data leaders in the financial sector.
London
September 20 - 21, 2023
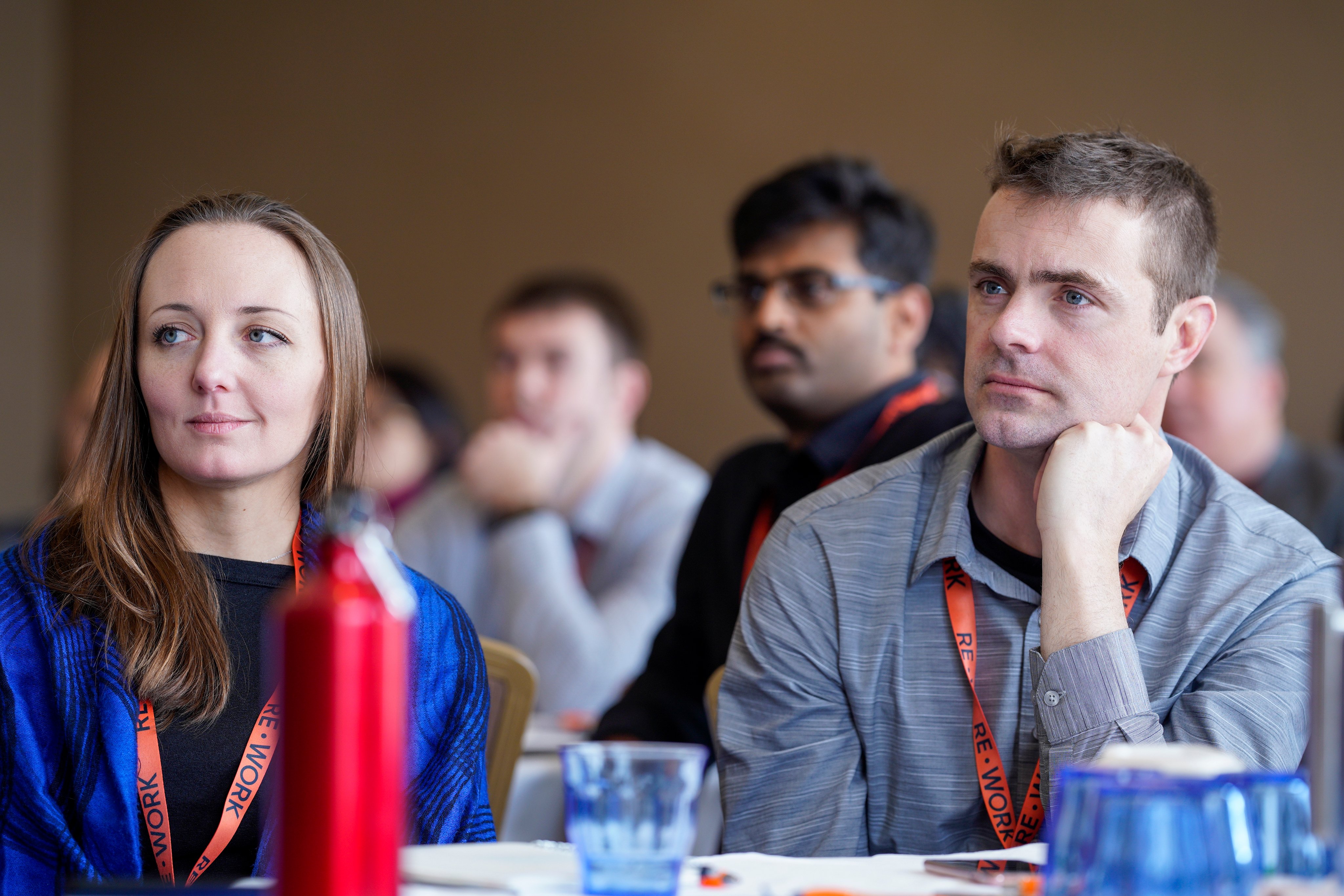
CDAO FS Frankfurt
Frankfurt
September 27 - 28, 2023
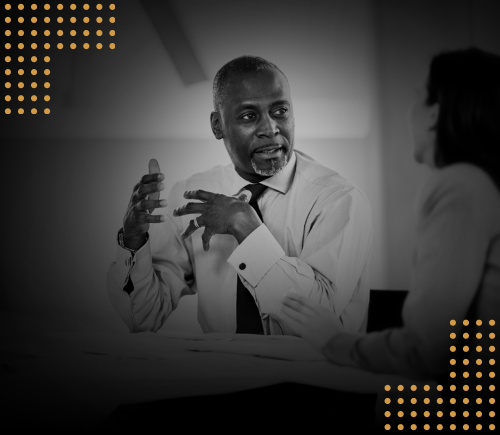
CISO Executive Network
An invite-only networking forum for the leading minds in infosec
12 Aug 2024 | Sydney CBD
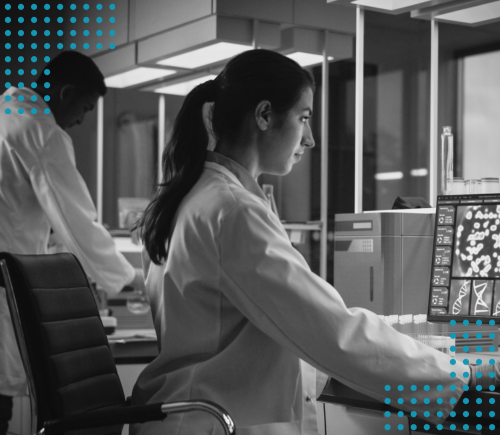
Data & Analytics in Healthcare
13 Mar 2024 | Grand Hyatt Melbourne
.png)
CDAO Malaysia
15 Oct 2024 | CBD
.png)
CISO Perth
Join prominent InfoSec leaders to get inspired, share intelligence and rekindle relationships
16 Apr 2024 | Perth Convention & Exhibition Centre
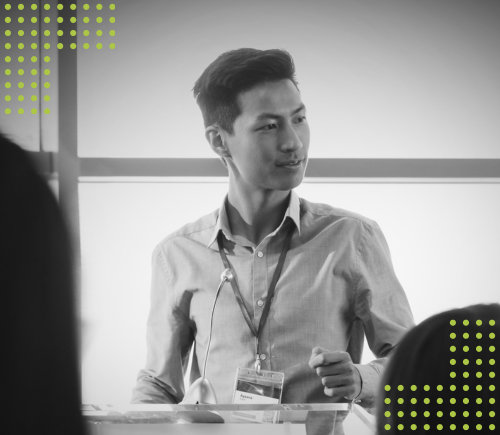
CDAO Singapore
The cross-industry exchange for all data and analytics leaders
16-17 Apr 2024 | Equarius Hotel Sentosa
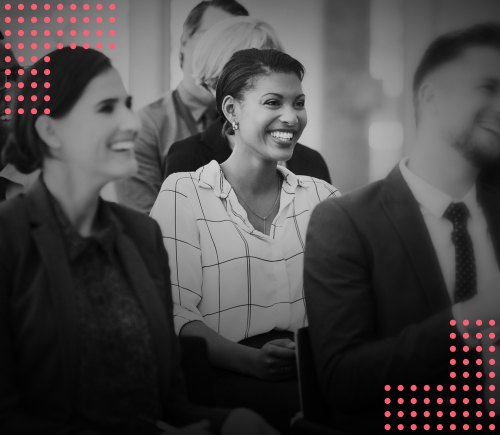
CISO Melbourne
16-17 July 2024 | Crown Promenade
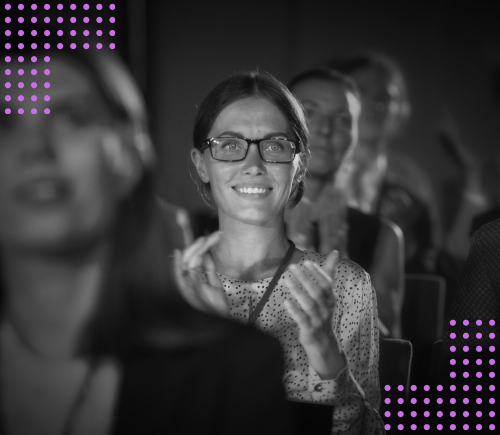
DevSecOps Melbourne
Co-located with CISO Melbourne 2024
17 July 2024 | Crown Promenade
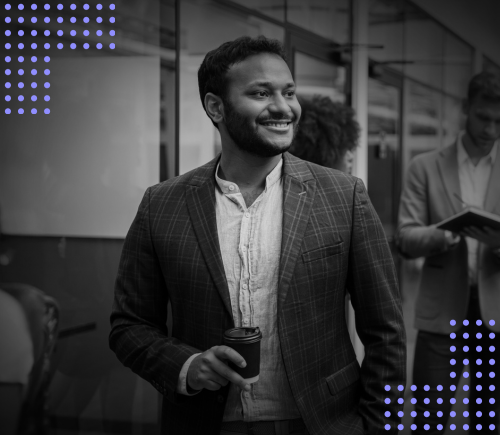
Cloud Security Melbourne
Co-located with CISO Melbourne 2024
17 July 2024 | Crown Promenade
.png)
Data Architecture Singapore
17 Sep 2024 | Singapore
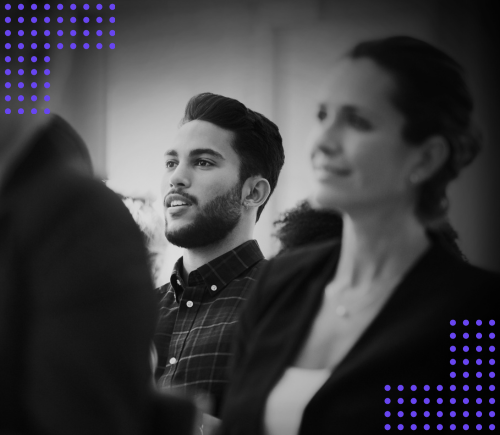
CISO New Zealand
19-20 Nov 2024 | Grand Millennium
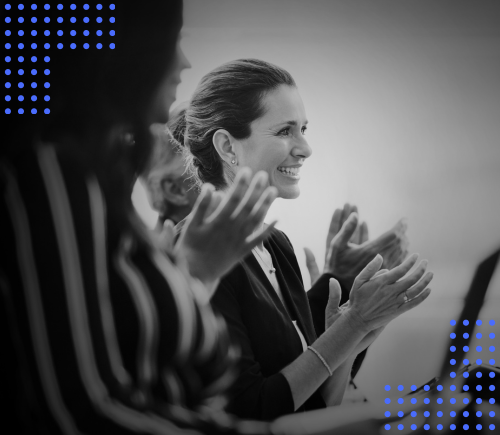
CDAO Melbourne
Connecting you to what's next in data
2-4 Sept 2024 | Crown Promenade Hotel
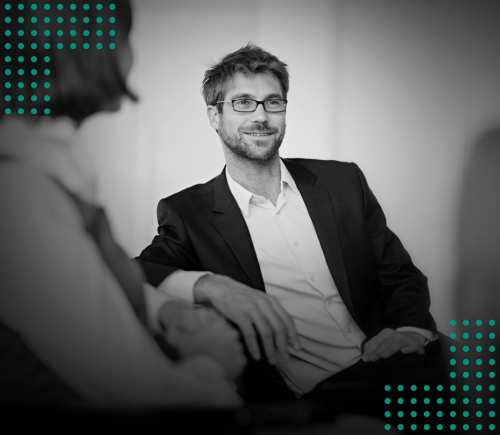
Data Architecture Melbourne
Building and maintaining flexible and scalable data architectures
20 June 2024 | Grand Hyatt
.png)
CISO Singapore
20-21 Aug 2024 | Equarius Hotel Sentosa
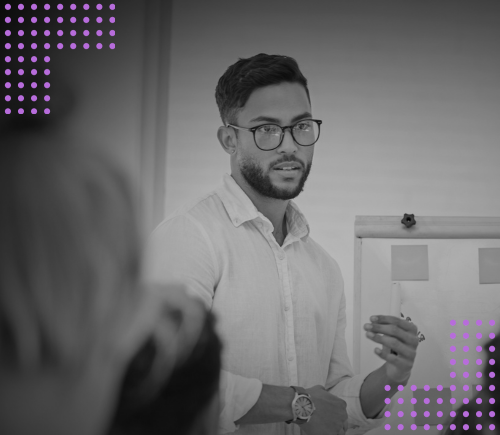
CISO Sydney
20-21 Feb, 2024 | Royal Randwick Racecourse
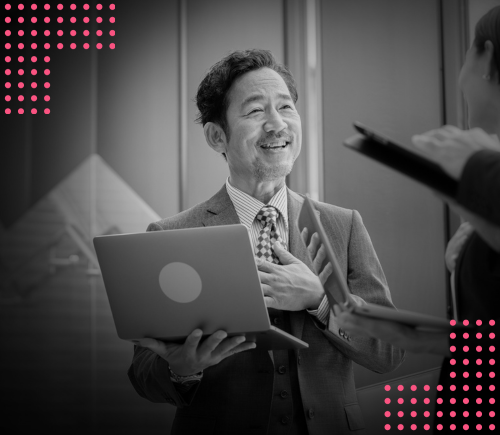
Cloud Security Singapore
21 Aug 2024 | Equarius Hotel Sentosa
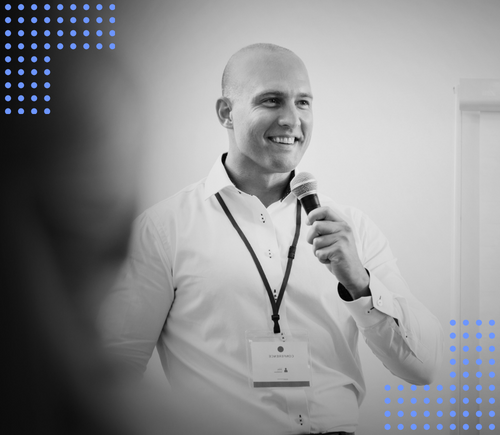
DevSecOps Sydney
21 Feb, 2024 | Royal Randwick Racecourse
.png)
CDAO Perth
22 Oct 2024 | Perth Convention Centre
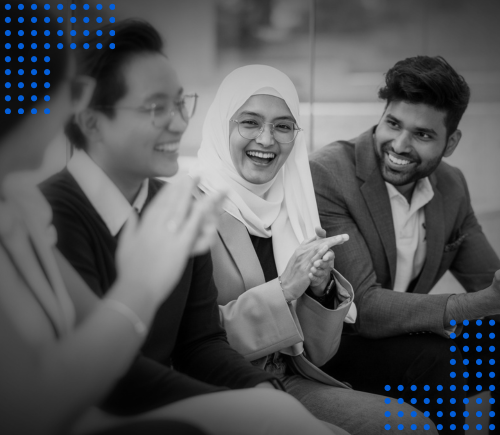
CDAO Indonesia
23 July 2024
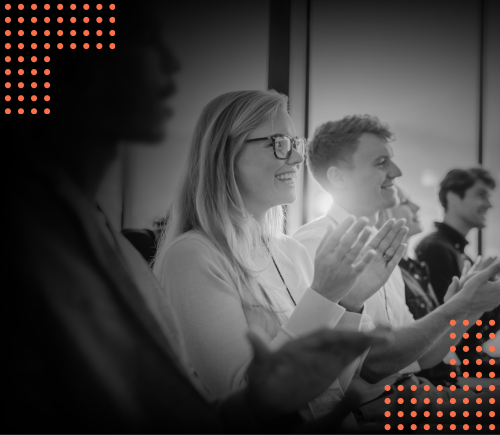
CISO Brisbane
Join prominent InfoSec leaders to get inspired, share intelligence and rekindle relationships
27 Aug 2024 | Hilton Brisbane
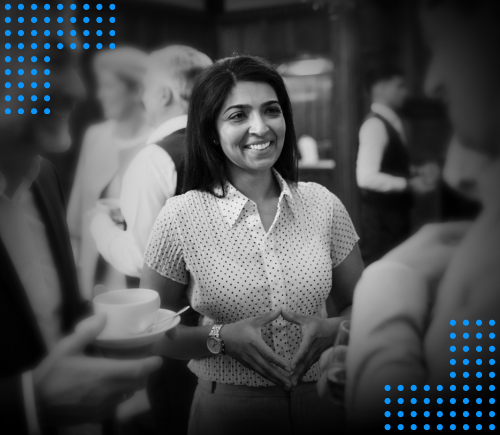
Data Architecture Sydney
27 June 2024 | Sydney Central Hotel
.png)
CDAO Brisbane
27-28 Feb 2024 | Hilton Brisbane
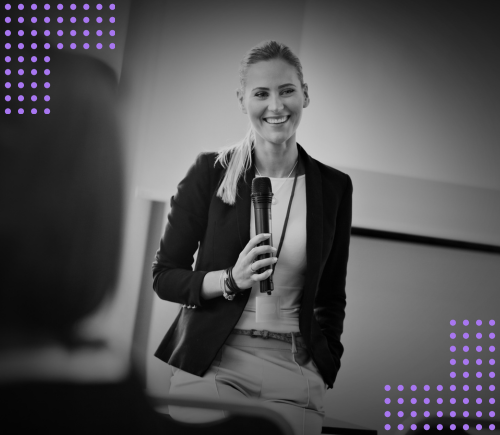
Modern DevOps Sydney
29 May 2024 | Sydney Central Hotel
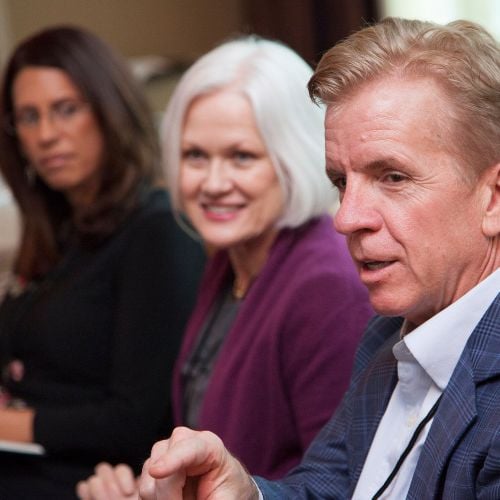
CISO Indonesia
29 Nov 2023 | Pullman Thamrin Jakarta
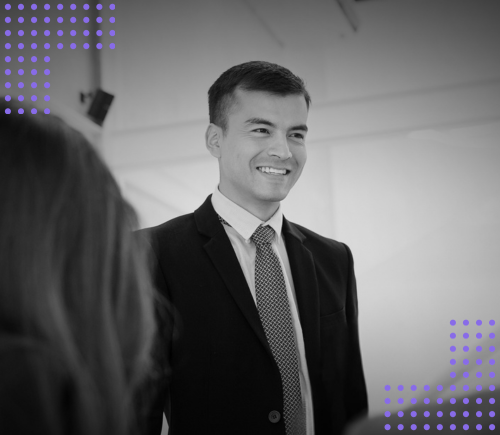
CISO Malaysia
30 Jan 2024 | Pullman Kuala Lumpur City Centre Hotel
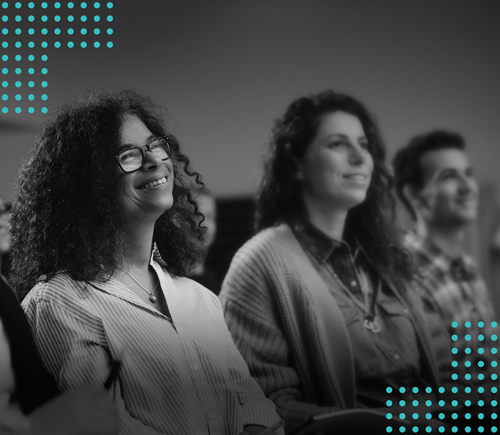
CDAO New Zealand
Connecting you to what's next in data
5-6 November 2024 | Grand Millennium Hotel
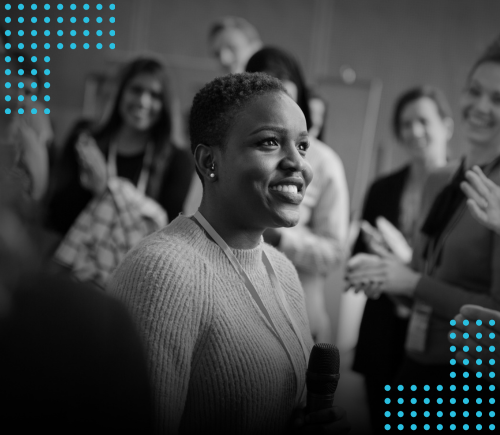
CDAO Sydney
Connecting you to what's next in data
7-8 May, 2024 | Royal Randwick Racecourse
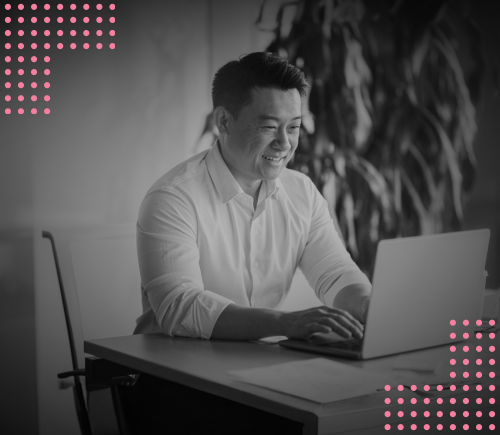
AI in Cyber Online
Put security at the core of your AI strategy
8 May 2024 | Online | 12 PM AEST | 10 AM SGT
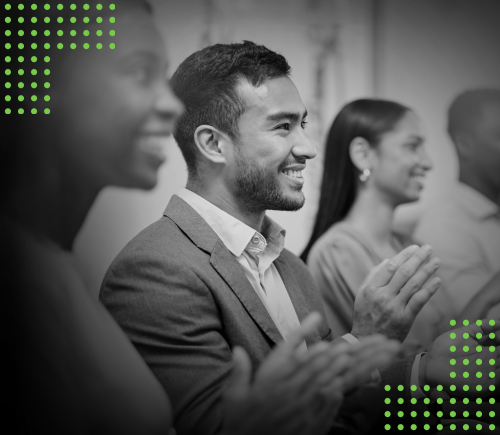
Data Architecture New Zealand
9 Apr 2024 | Hilton Auckland
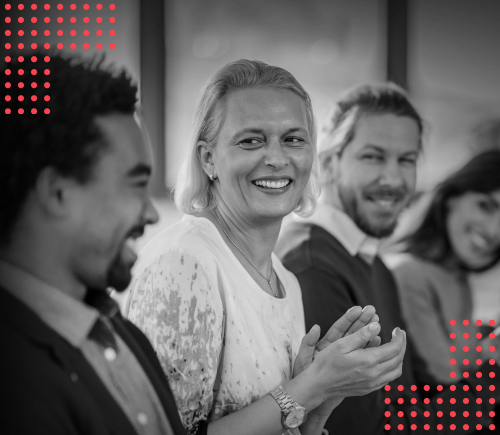
Modern DevOps Melbourne
9 October 2024 | Melbourne
.png)
CDAO Germany
Putting Data at the Heart of Business, Driving Value in Uncertain Times
Munich
April 17-18, 2024
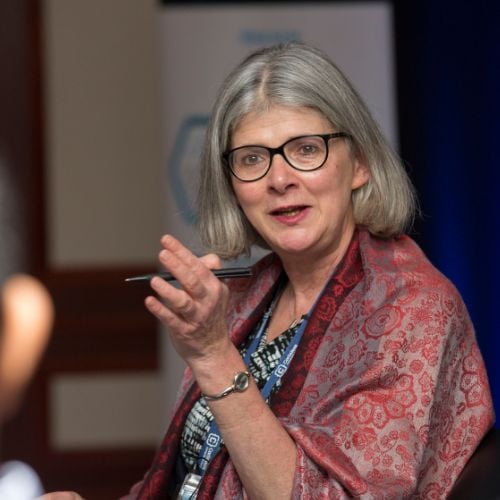
The West Coast Data & Analytics Event
Chief Data & Analytics Officer Spring (CDAO Spring)
San Francisco
April 23-24, 2024
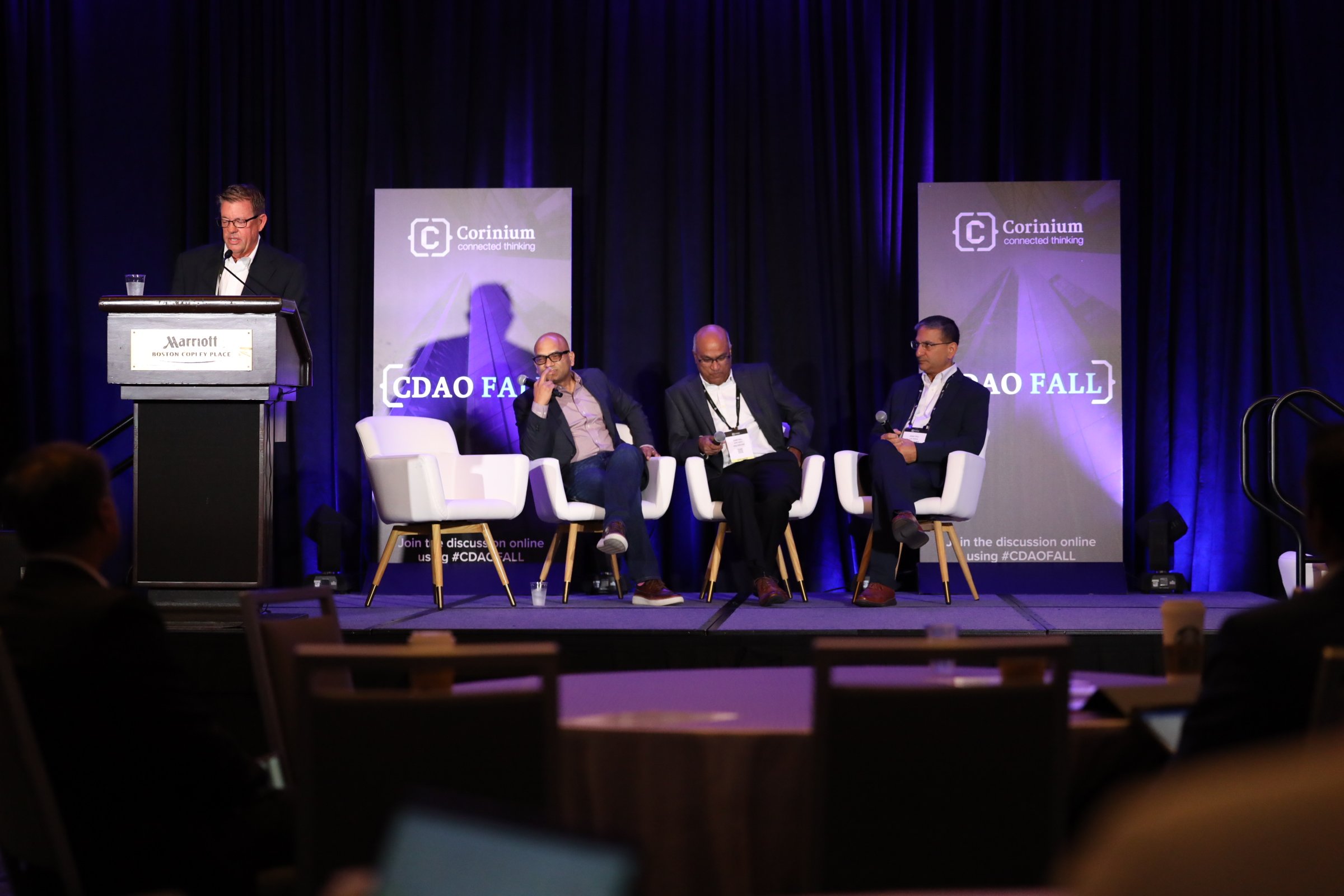
CDAO Chicago
Reconnecting Chicago's Data & Analytics Leaders In-Person to Accelerate Their Data Transformation Strategies
Chicago
August 7-8, 2024
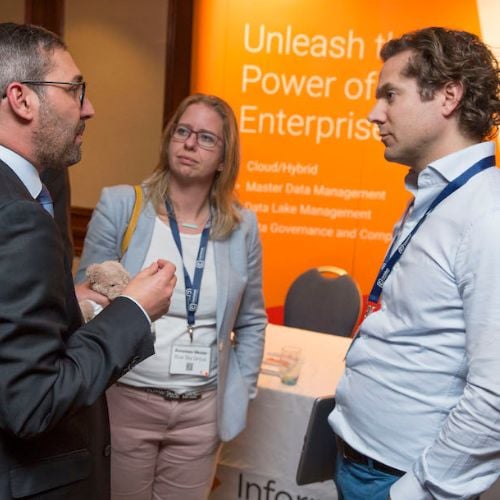
CDAO APEX West
The West Coast's Leading Senior Executive Event for Data & Analytics Leaders
Tempe
December 5-6, 2023
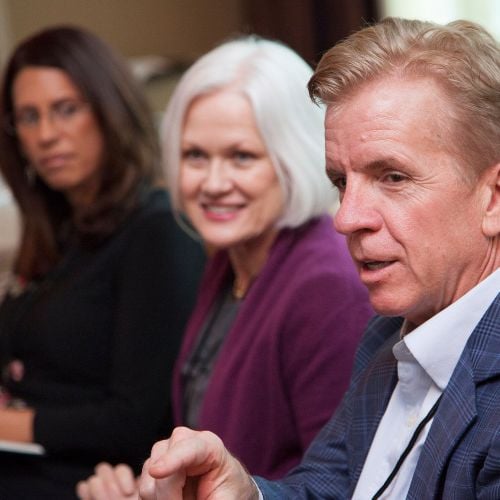
CDAO UK
Join the UK's most senior data & analytics event
London
February 12-13, 2025
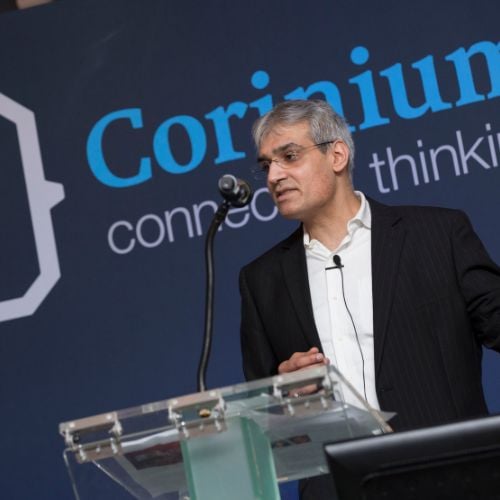
CDAO Financial Services
Accelerate Data-Driven Growth and Innovation
New York
February 25-26, 2025
.png)
Data & Analytics Live
The best of Data & Analytics in a virtual platform
North America
January 18-19, 2023
.png)
Data & Analytics Live
The best of Data & Analytics in a virtual platform
North America
July 17, 2024
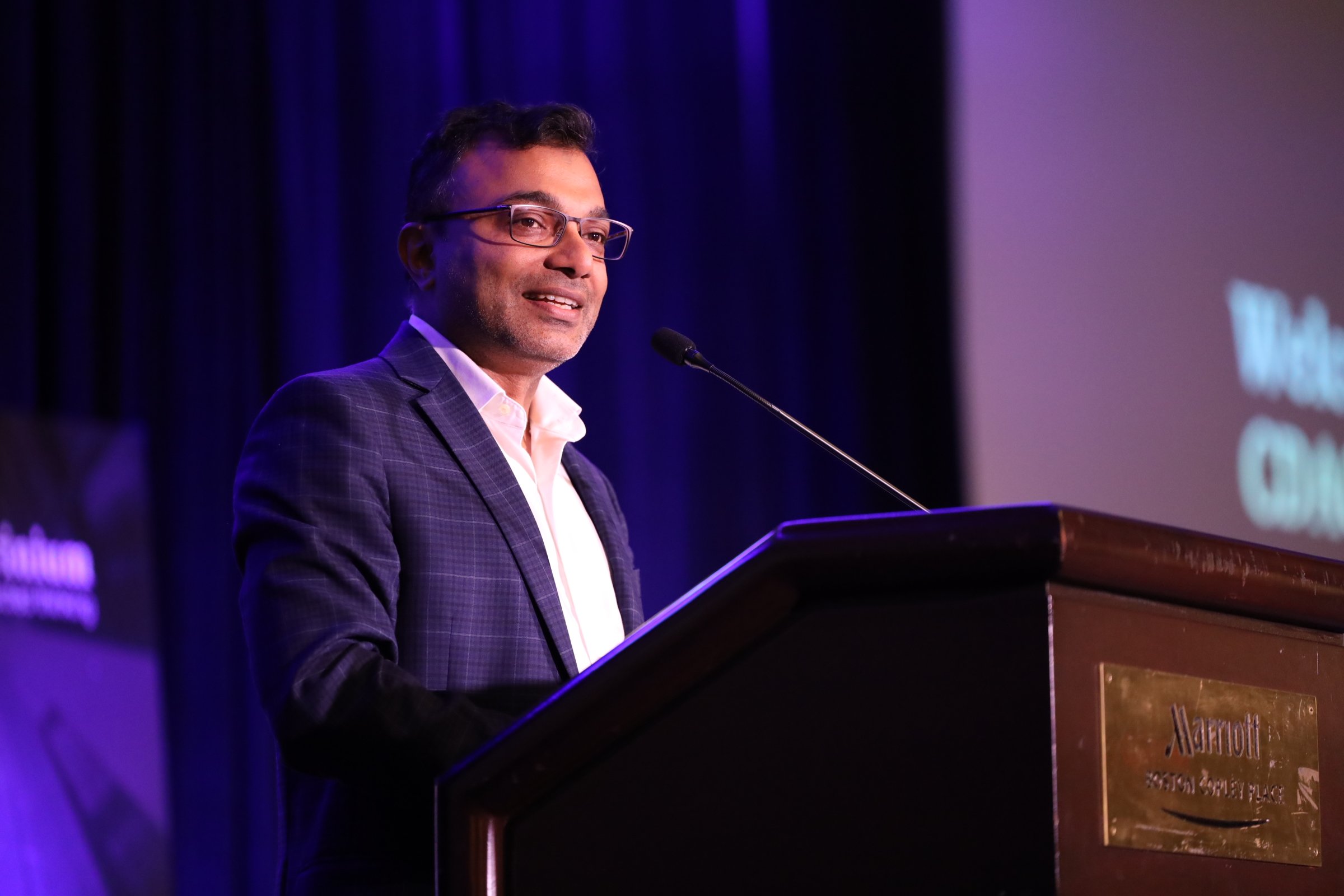
CDAO Canada Public Sector
The Premier Event for Canada's Public Sector Data & Analytics Leaders
Ottawa
June 18-19, 2024
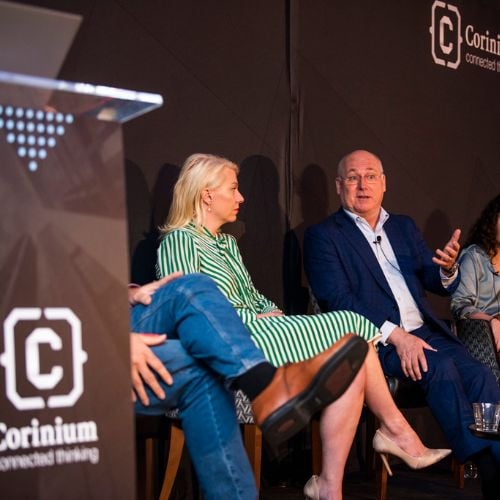
CDAO France
Conçu pour les leaders des données stratégiques et de l'analyse des plus grandes marques françaises, CDAO France sera le mélange parfait d'informations pratiques et de réseautage de haut niveau.
Paris
Mai 29 2024
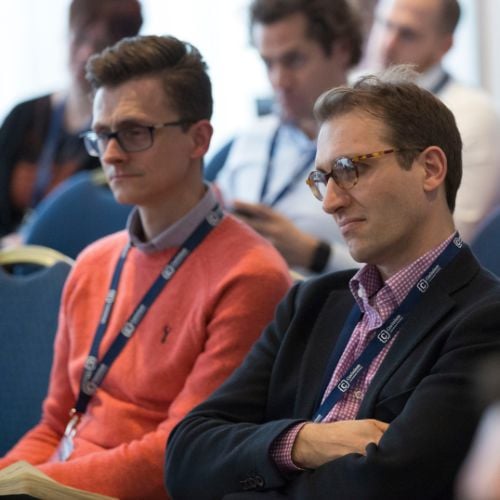
CDAO Canada
Transforming the Data and Analytics Community Through Innovation to Deliver Enhanced Business Value
Toronto
March 26-27, 2024
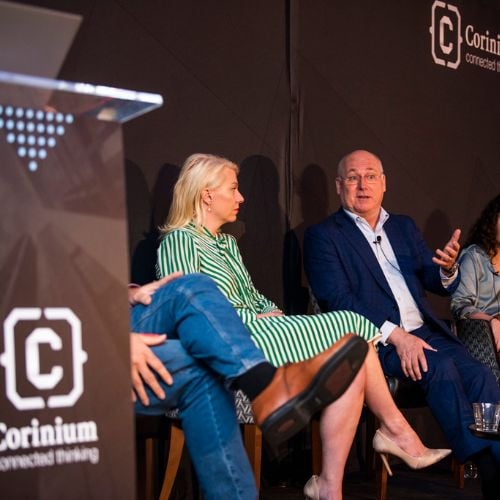
CDAO APEX Financial Services
Finance and Banking's Leading Senior Executive Event for Data & Analytics Leaders
Charlotte
May 14-15, 2024
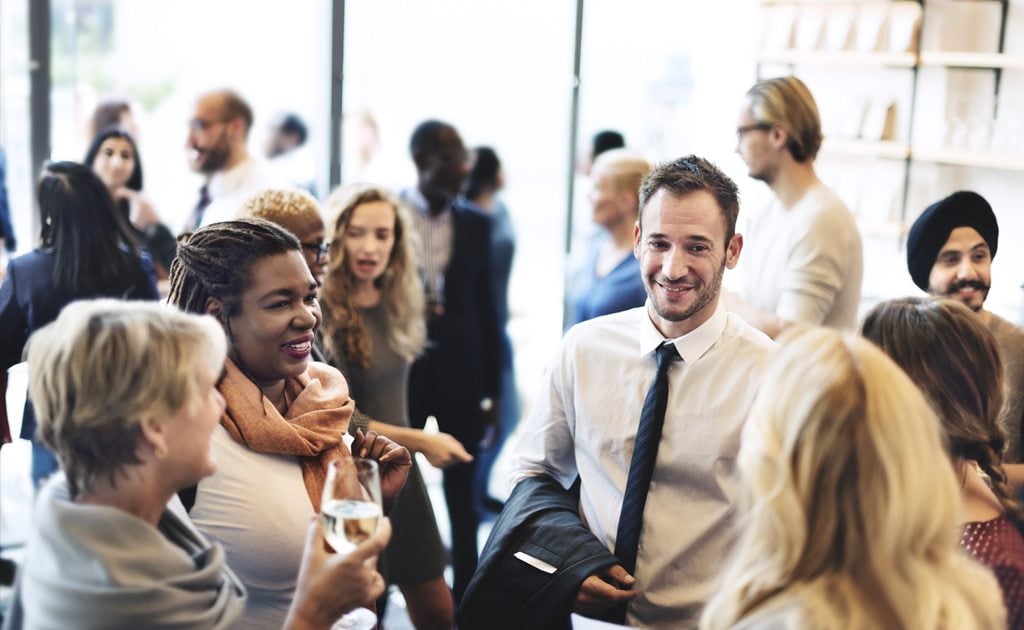
CISO Public Sector Online A/NZ
Reinforcing public sector cyber security resilience in Australia and New Zealand
Online
May 2, 2023
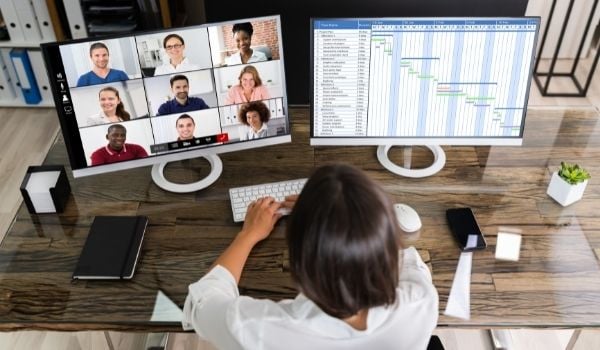
CISO ASEAN Online
Online
May 30, 2023
.png)
CDAO Nordics
Practical insights for data leaders
Stockholm
November 14, 2024
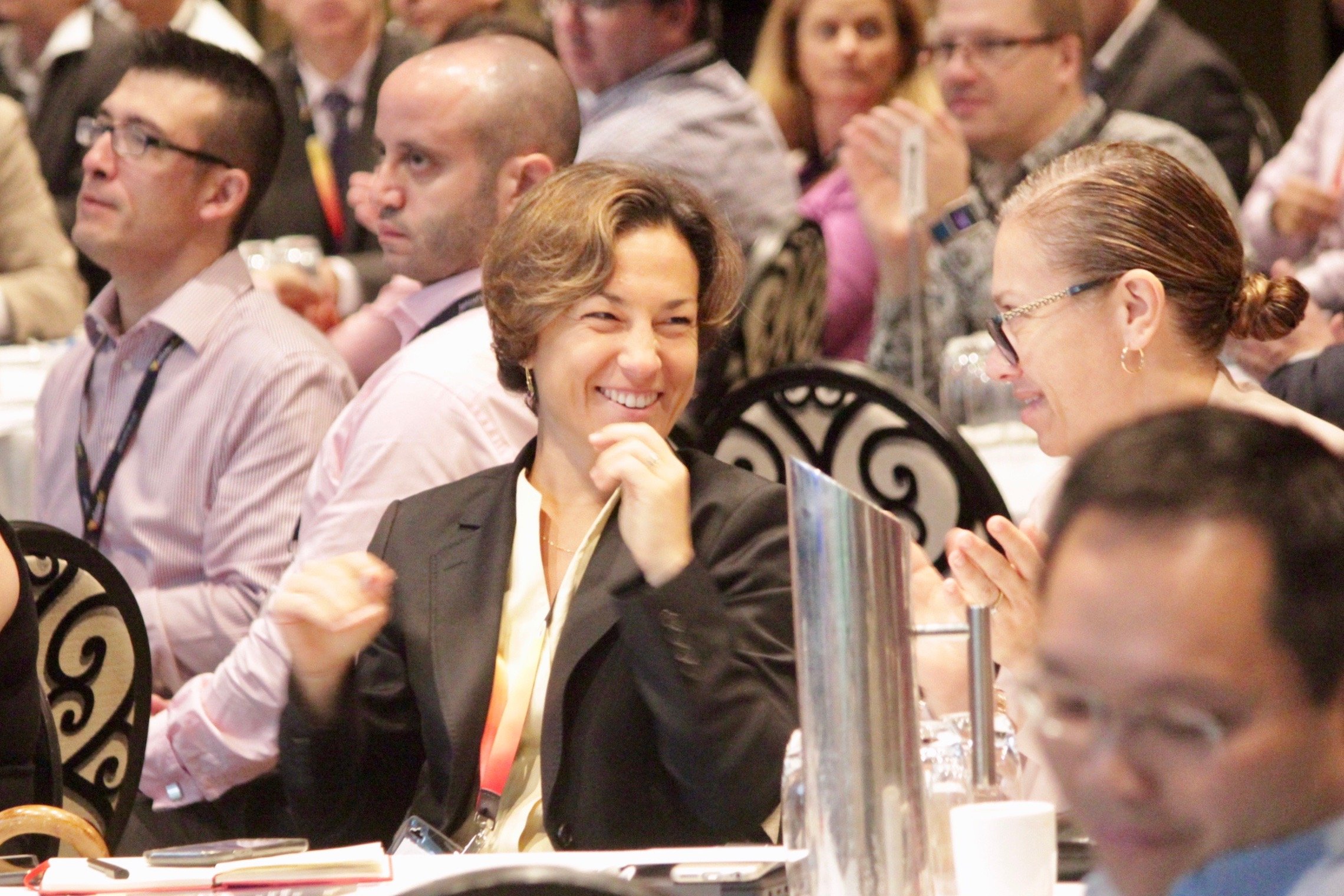
CDAO Europe 2024
Europe's Most Senior, Cross - Industry Data & Analytics Event
Amsterdam
November 7, 2024
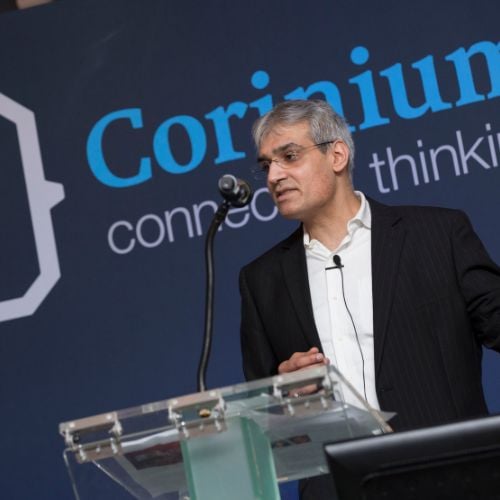
CDAO Fall
The Premier In-Person Gathering for Data & Analytics Leaders in North America
Boston
October 15-17, 2024
.png)
CDAO Frankfurt
Putting Data at the Heart of Business, Driving Value in Uncertain Times
Frankfurt
October 31, 2024
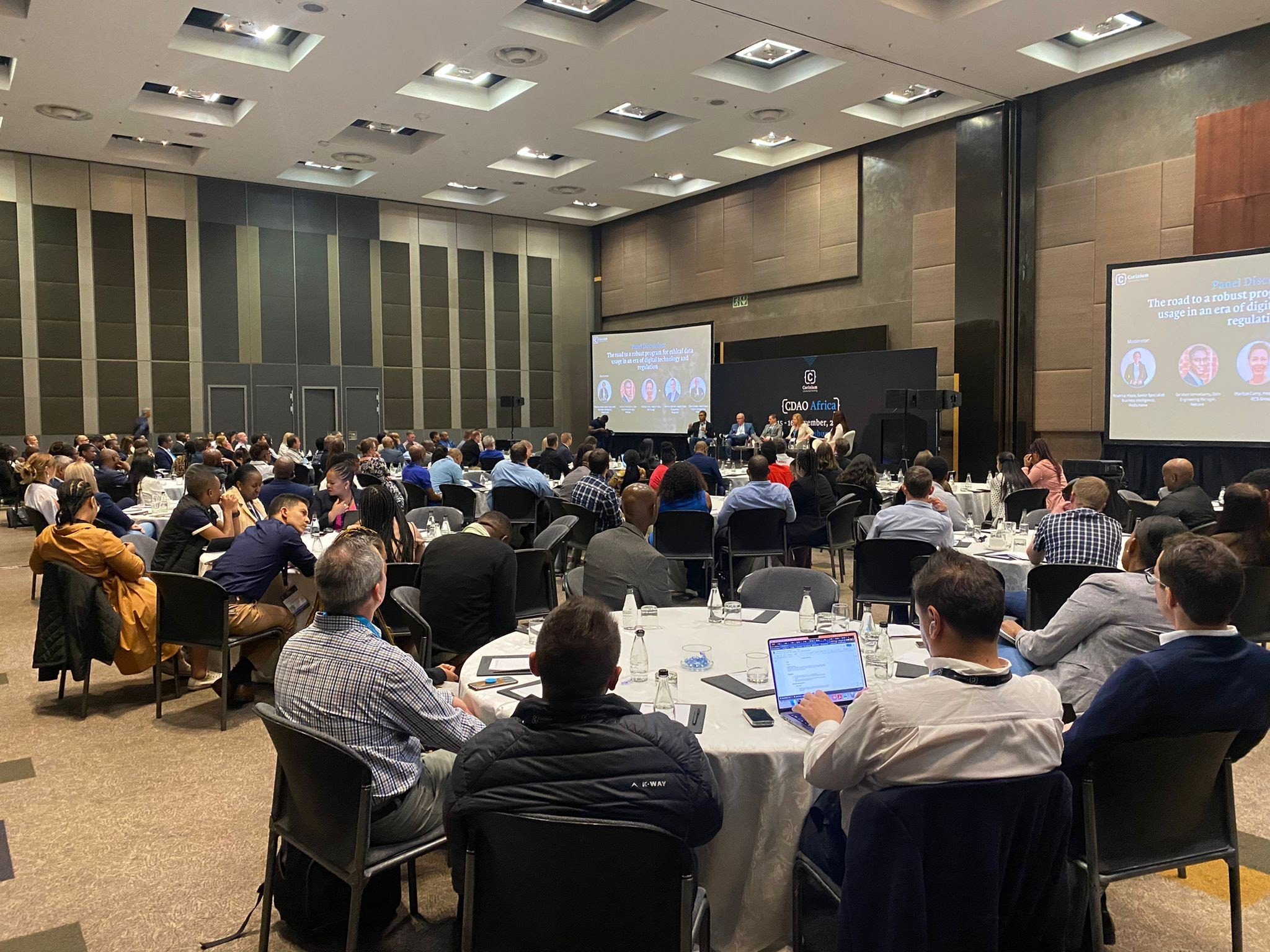
CDAO Financial Services & Insurance UK 2024
Accelerate Data-Driven Growth and Innovation within Financial Services and Insurance
London
September 11 - 12, 2024
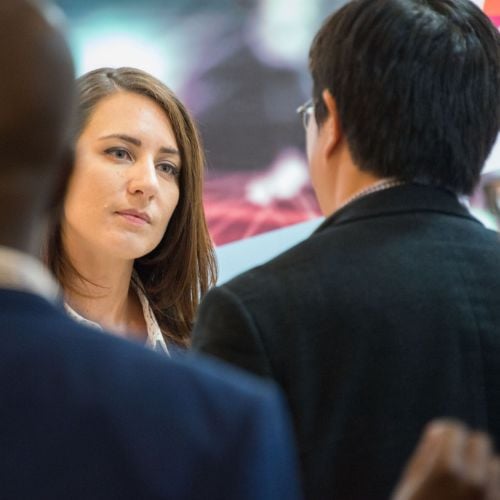
CDAO Government
The Longest Running Event for Federal, State, and Local Government Data & Analytics Leaders
Washington D.C.
September 18-19, 2024 | Convene