All our events
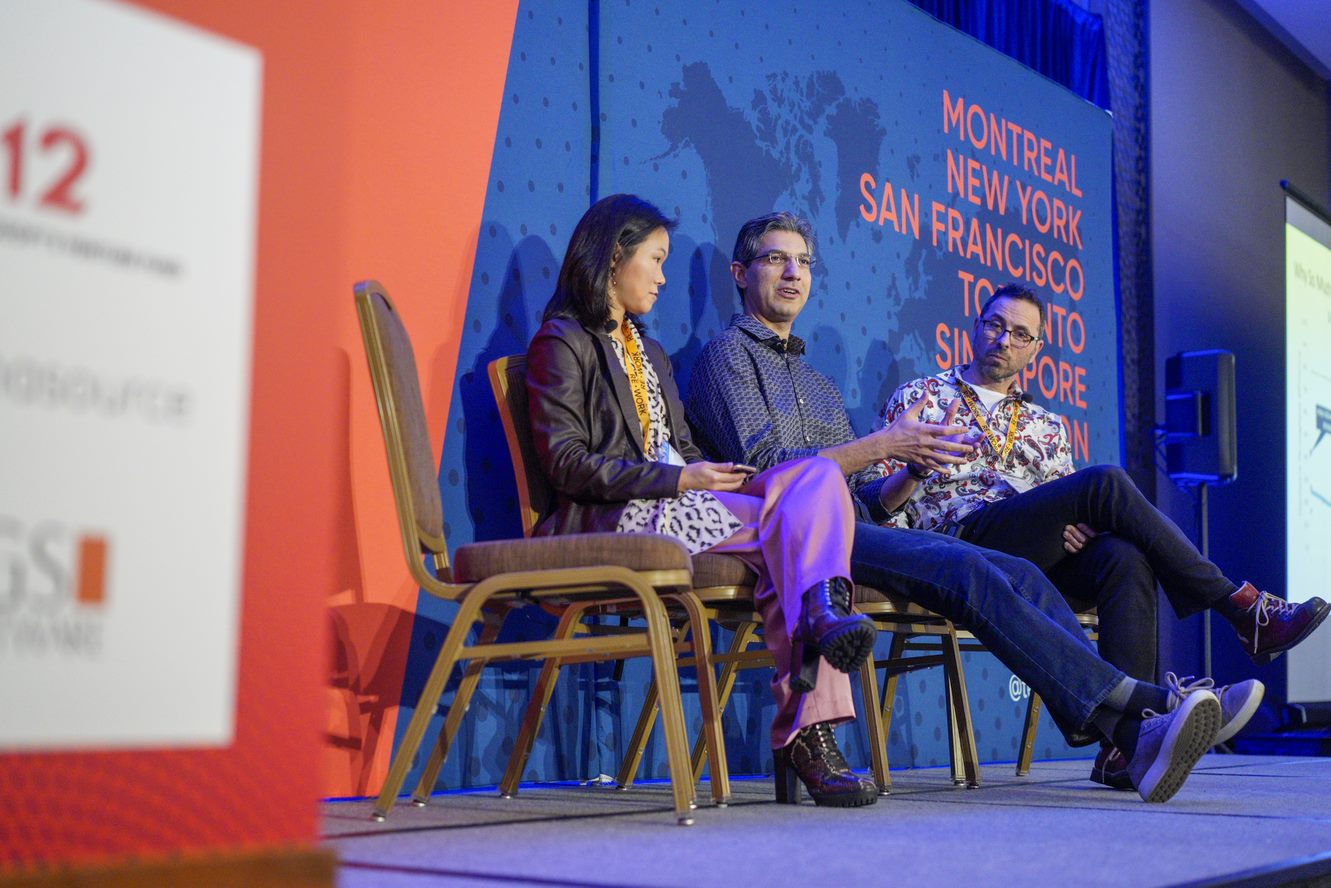
Conversational AI Summit
This summit will bring together experts in the field of conversational AI to discuss the latest advancements, challenges, and best practices in designing, developing, and deploying conversational AI systems. The event will feature keynote speakers, workshops, and panel discussions covering topics such as natural language processing, chatbot development, voice assistants, and the ethical considerations of conversational AI.
London
16-17 May 2023
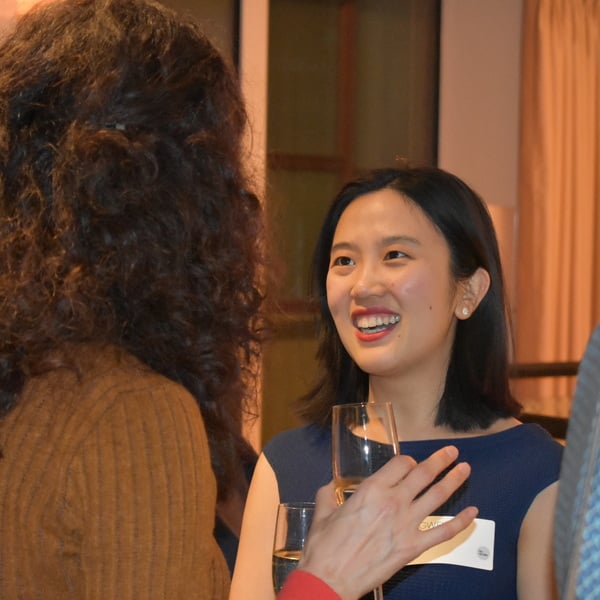
Women In AI & Data Reception
Join us for an evening of thought-provoking discussions, presentations and a networking reception. Support the achievements and advancement of women within the AI industry. The evening is open to all genders!
London
24 January, 2023
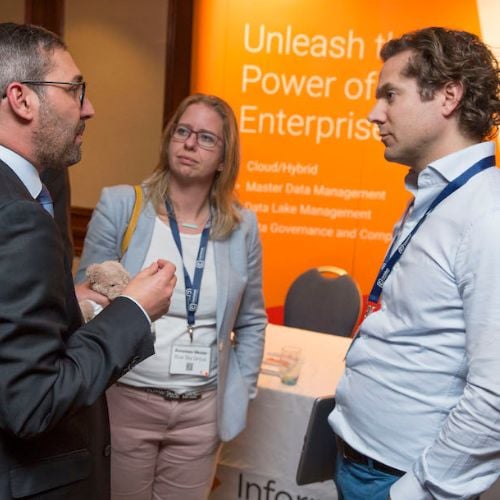
Chief AI Officer Summit UK
The #1 Event in Europe Dedicated to Chief AI Officers and AI Practitioners
London
25 February, 2026
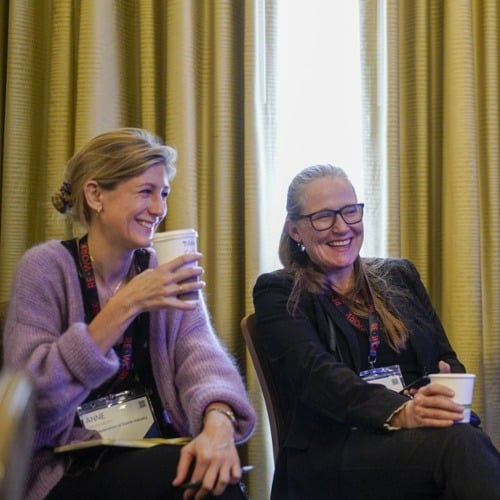
AI in Finance Summit London
Explore the latest AI tools & techniques and their application in the financial sector with data scientists, ML engineers, researchers & CTOs across the industry. Applications include wealth and portfolio management, retail banking, trading & payments innovation.
London
9 September 2025
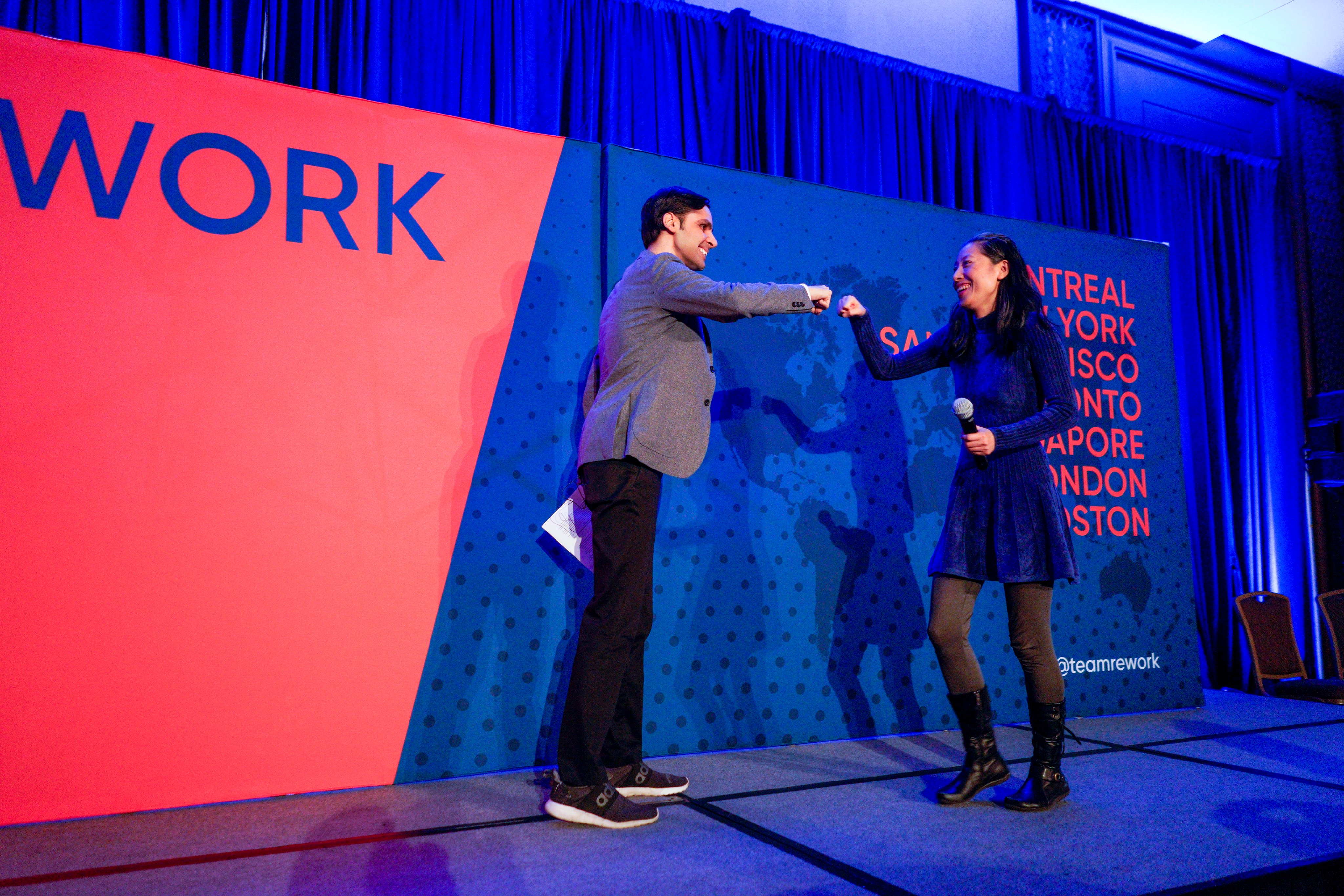
AI in Finance Summit New York
Explore the latest AI tools & techniques and their applications with top speakers from the banking, insurance, academia and the financial sector. CTOs, Directors, ML engineers, Data Scientists and Researchers will share their insights into recent breakthroughs in technical advancements and fintech applications across the industry.
New York
April 15-16, 2025
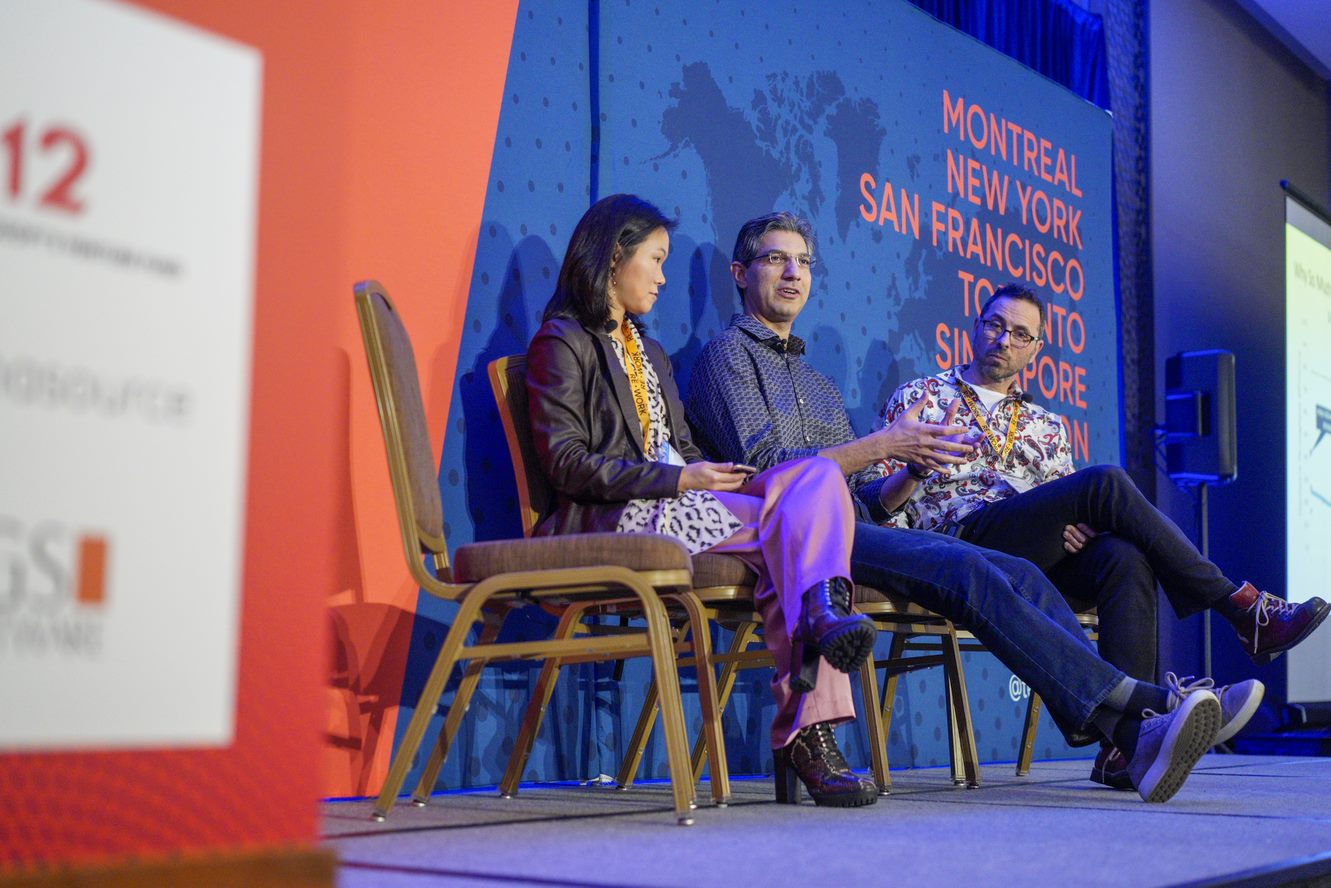
Chief AI Officer Summit
The Premier East Coast Event Dedicated to Chief AI Officers and AI Practitioners
New York
April 16, 2025
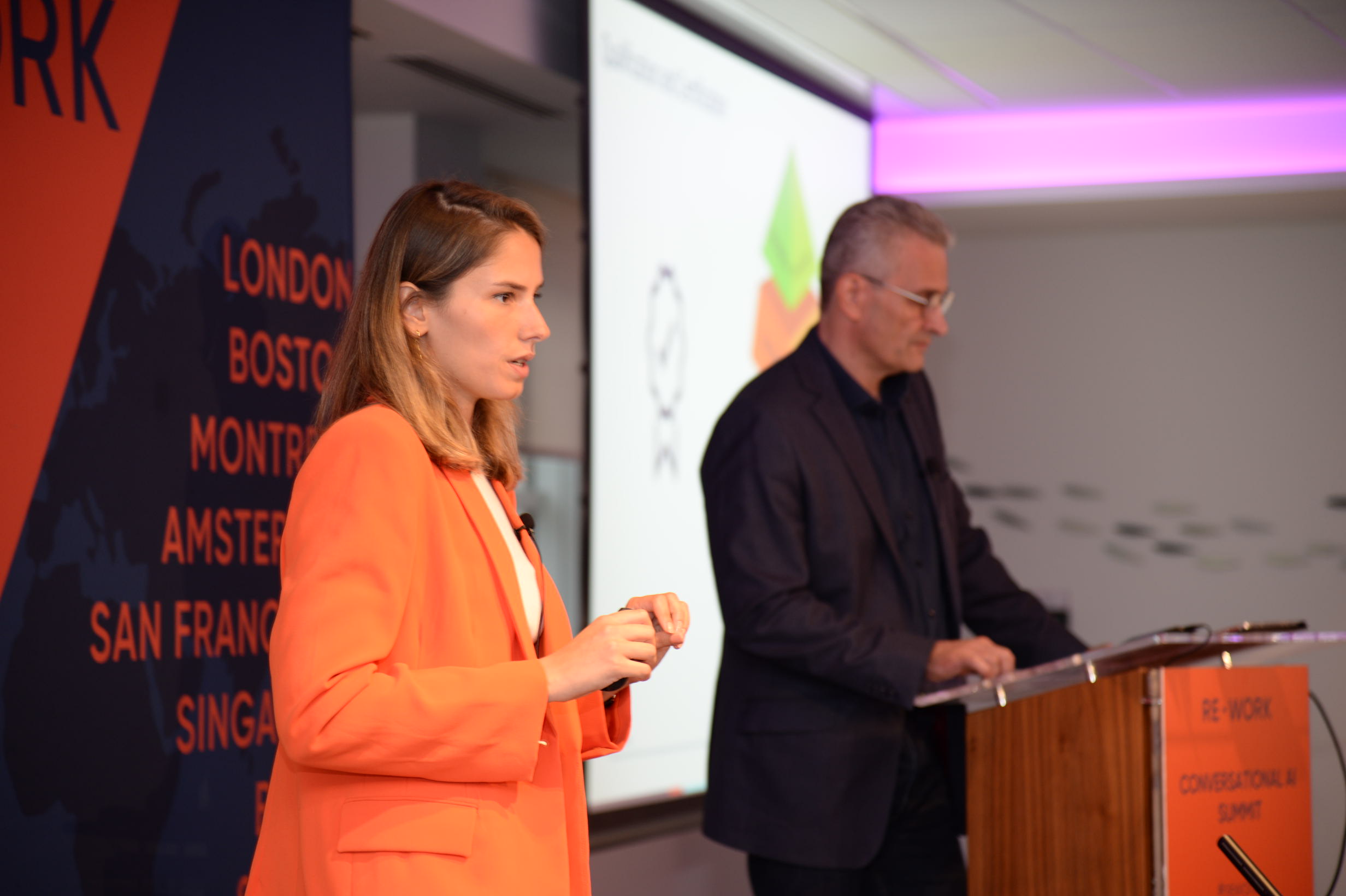
AI Summit West: Deep Learning & Advanced ML
Explore the latest breakthroughs from researchers in the field of Deep Learning and Advanced ML. Discover ways to overcome obstacles in developing and deploying deep neural networks as well as novel algorithmic advancements from leading academics and industry professionals.
San Jose
February, 2026
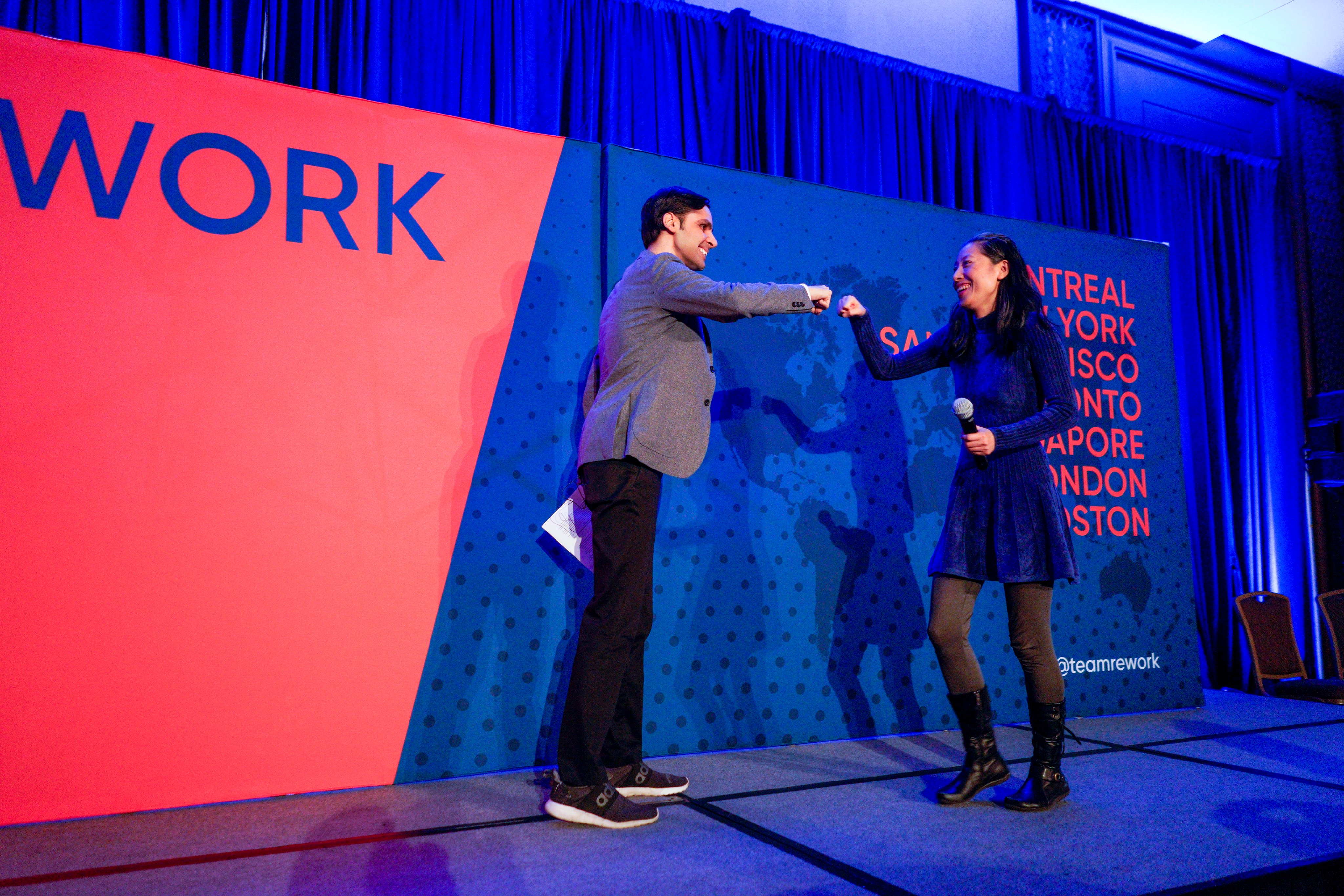
AI in Healthcare & Pharma Summit
This summit will showcase the latest advancements in AI technologies and their applications in healthcare. Speakers will discuss the potential of AI to improve patient outcomes, streamline clinical workflows, and address healthcare challenges such as the COVID-19 pandemic. The conference will also address the ethical considerations of AI in healthcare, such as data privacy and bias, and how to ensure that AI technologies are developed and deployed in a responsible manner.
Boston
November 18-19, 2025
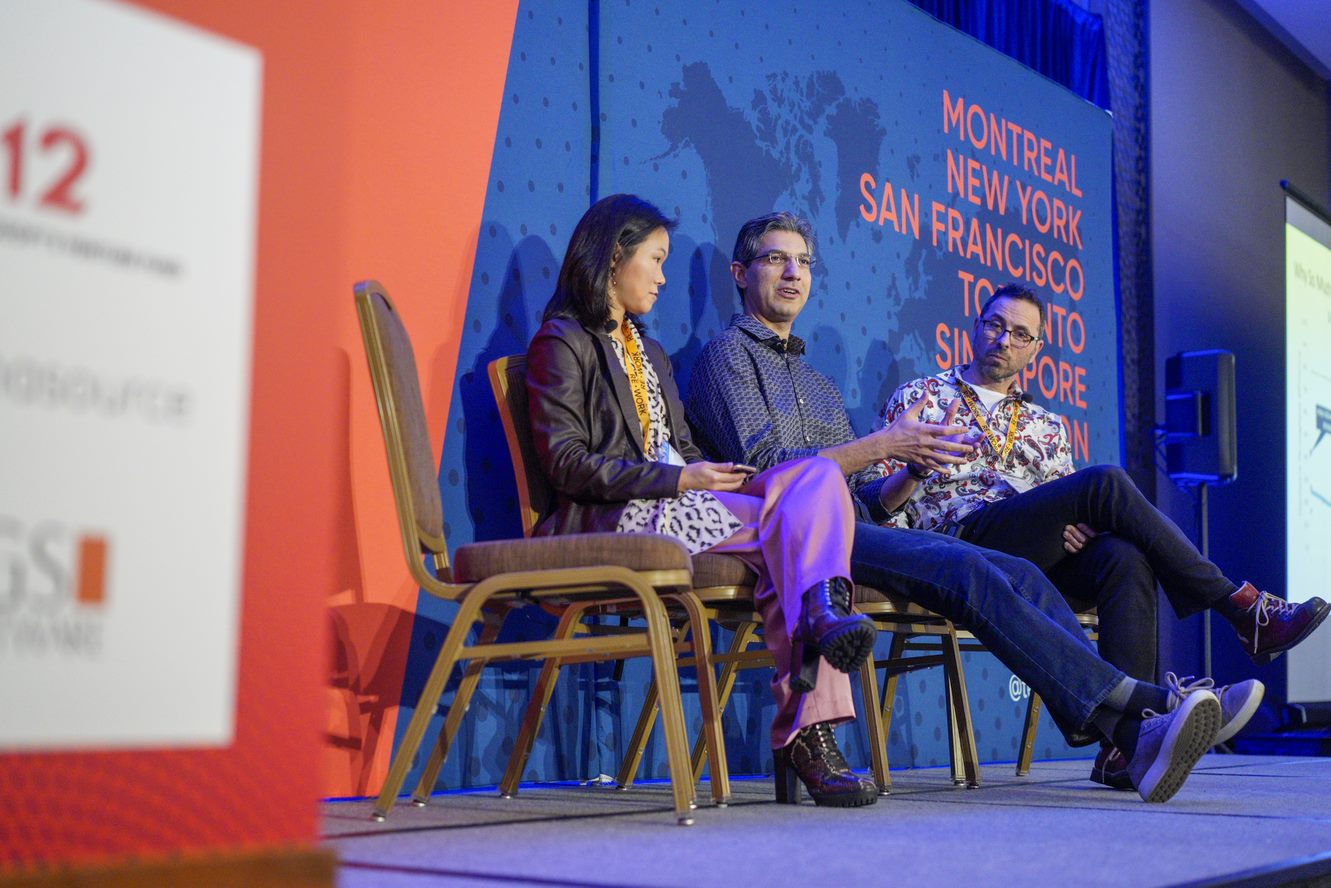
Applying Next Level Deep Learning and Advanced Machine Learning
Enterprise AI Summit Canada
Applying Next Level Deep Learning and Advanced Machine Learning
Toronto
October 22-23, 2024
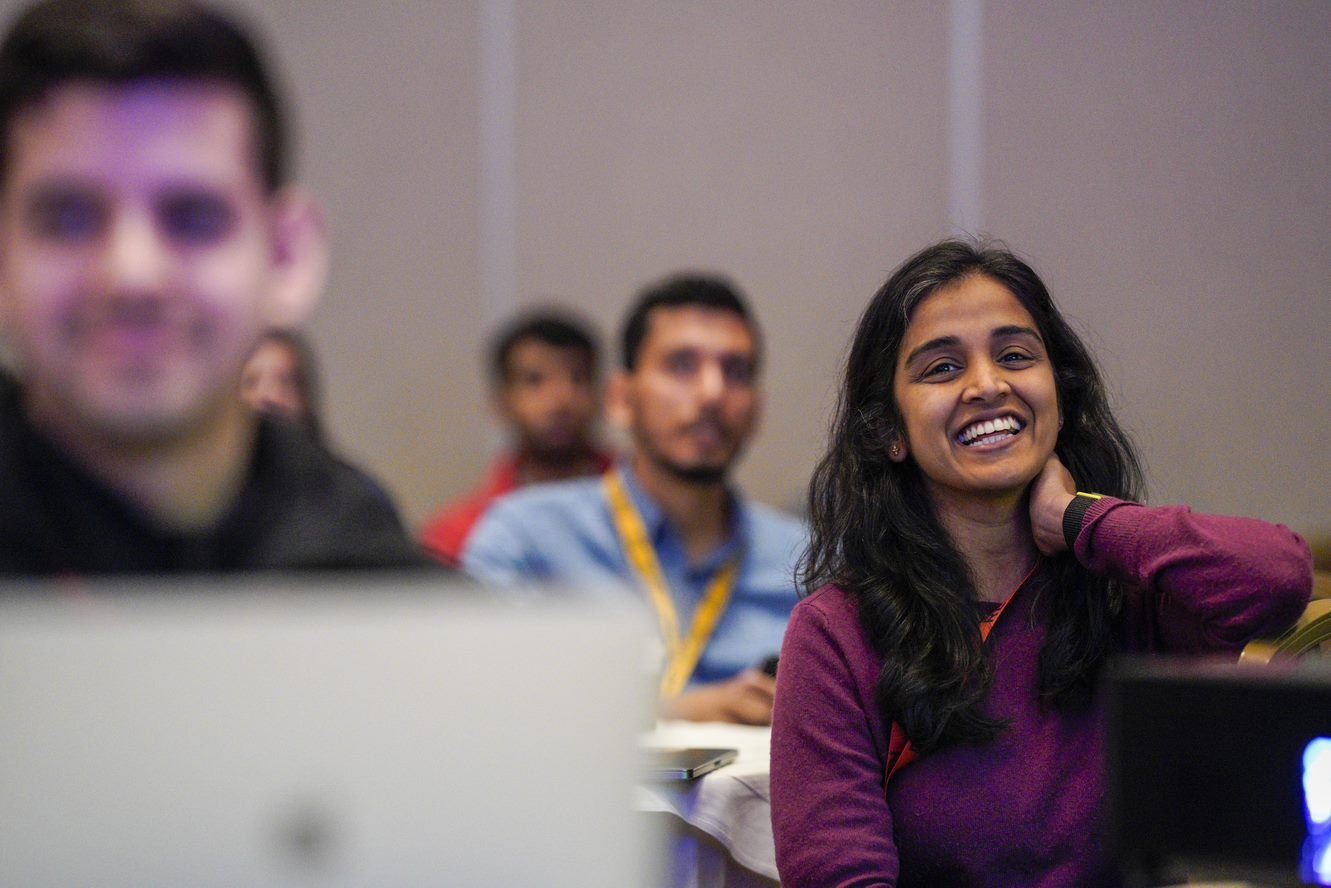
CDAO FS London
Join the UK's most senior event for data leaders in the financial sector.
London
September 20 - 21, 2023
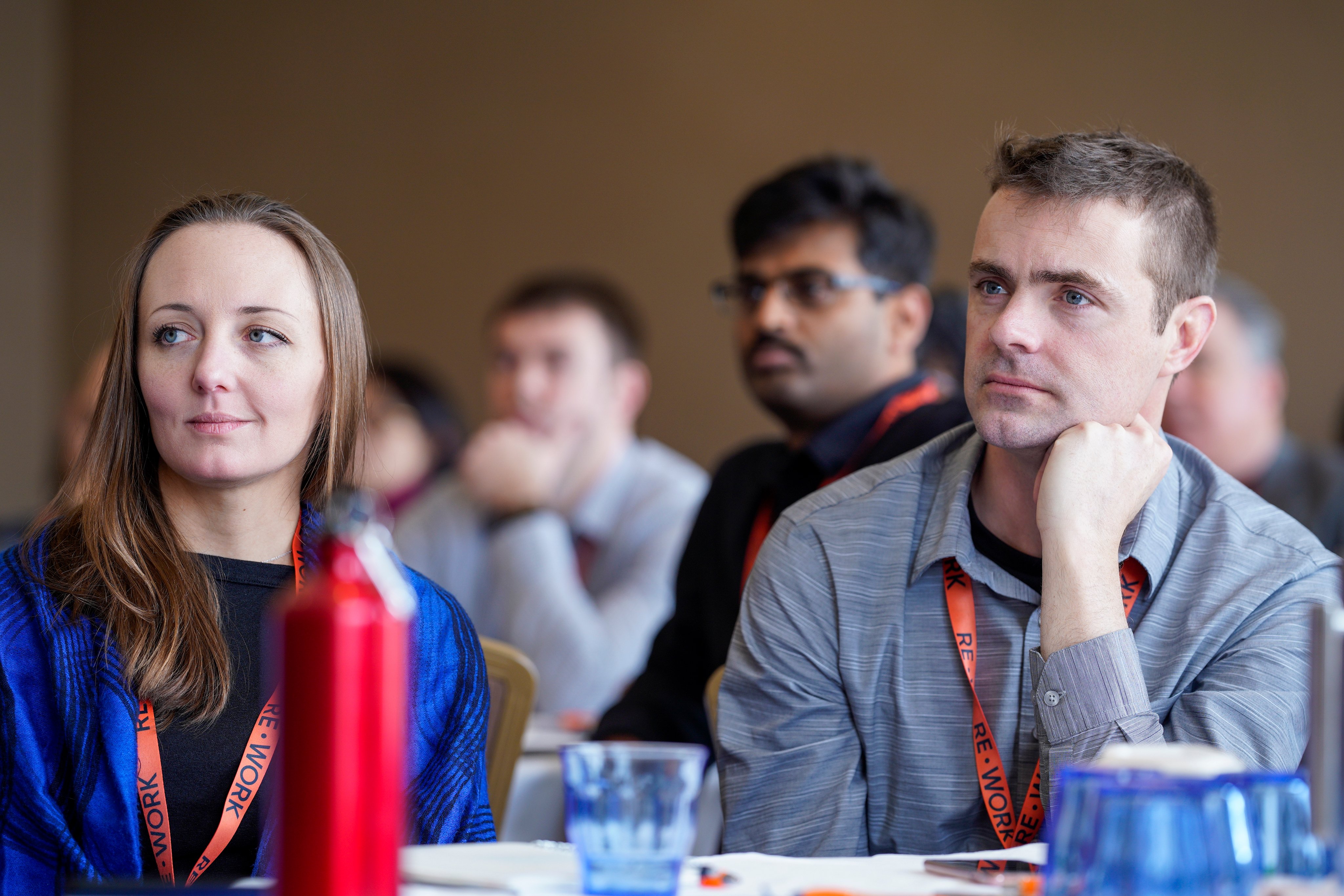
CDAO FS Frankfurt
Frankfurt
September 27 - 28, 2023
.png)
CISO Perth
Join prominent InfoSec leaders to get inspired, share intelligence and rekindle relationships
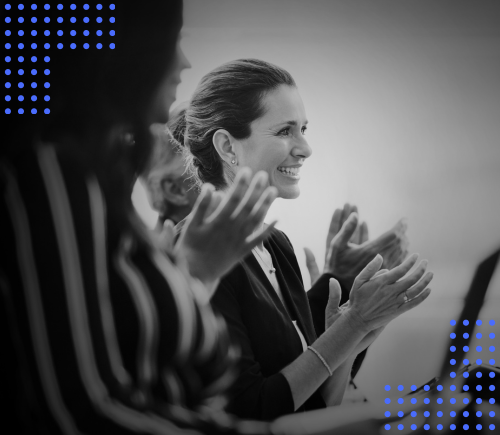
CDAO Melbourne
Connecting you to what's next in data
10 - 11 Sept 2025
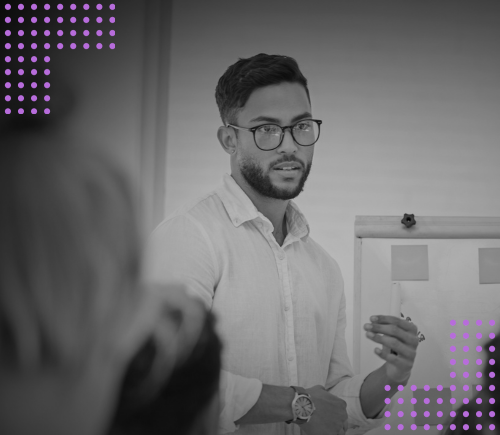
CISO Sydney
Sydney
10-11 Feb, 2026
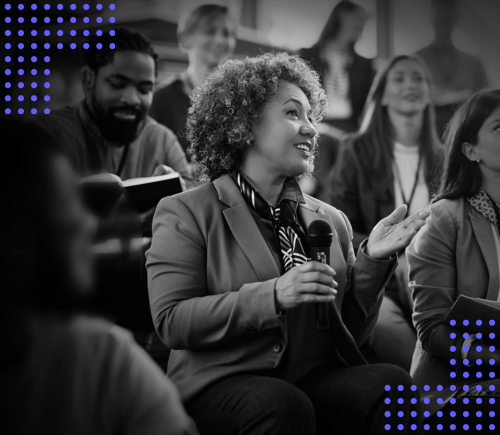
OT Security Sydney
11 Feb 2026
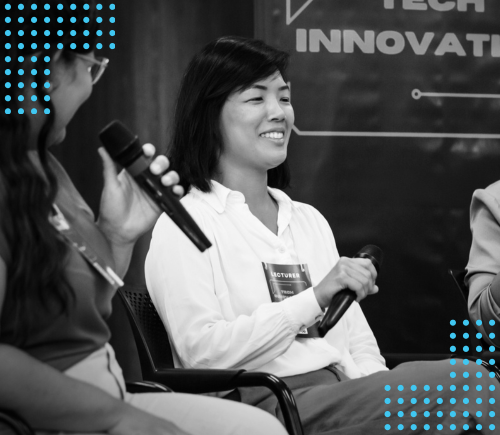
Enterprise AI Melbourne
Enterprise AI Melbourne brings together the trailblazers guiding AI adoption in Australian businesses.
11 Sept 2025
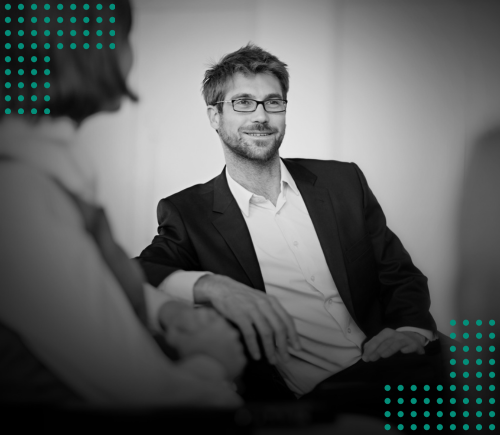
Data Architecture Melbourne
Join our exclusive gathering of top data architecture leaders, where bold ideas, strategic thinking, and powerful connections come together to shape the future of our industry.
11 Sept 2025
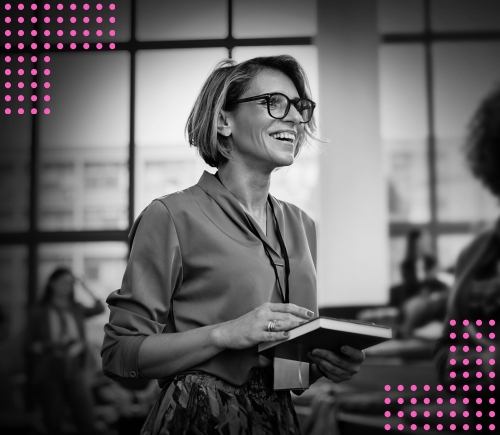
Cloud Infrastructure Melbourne 2025
Melbourne
12 August 2025
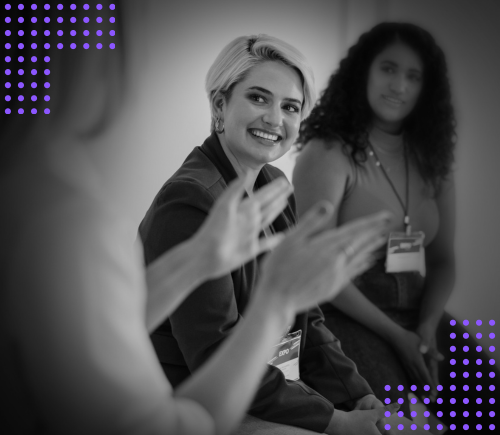
Cloud Security Sydney
12 Feb 2025
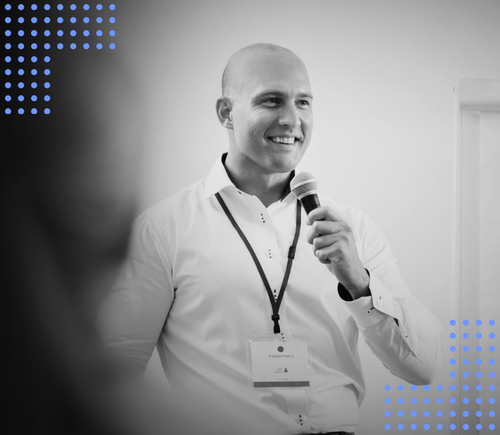
AppSec & DevSecOps Sydney
12 Feb, 2025
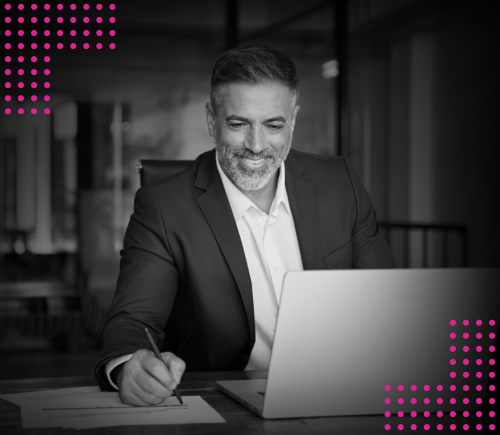
CISO FSI ANZ
12 Sept 2024 | Online | 10 AM AEST | 12 PM NZT
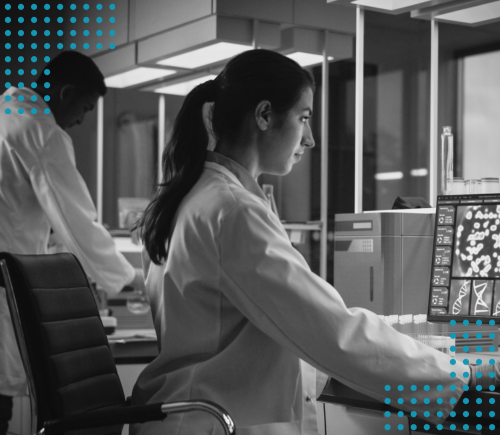
Data & Analytics in Healthcare
13 Mar 2024 | Grand Hyatt Melbourne
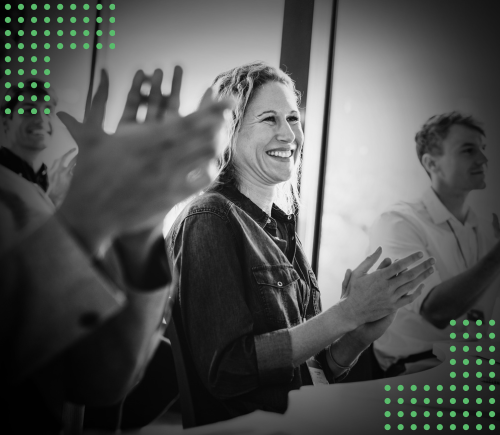
CDAO Perth
13 Oct 2025
-1.png)
CDAO FSI LatAm
"Conectando a los líderes en datos y analítica para servicios financieros y seguros de México y América Latina"
Ciudad de México
14 y 15 de octubre, 2025
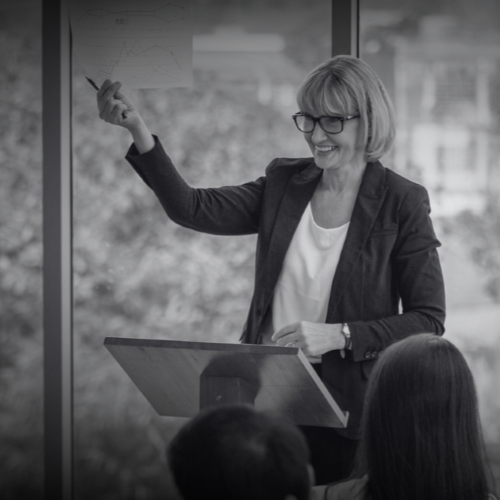
CDAO Financial Services Europe
Putting Data at the Heart of Business, Driving Value in Uncertain Times
Geneva
16 October 2025
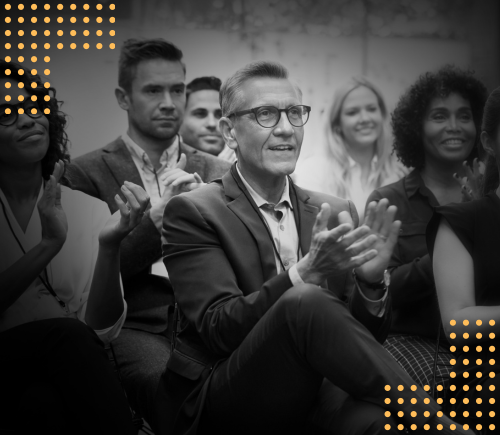
CISO Canberra
17 Sept 2025
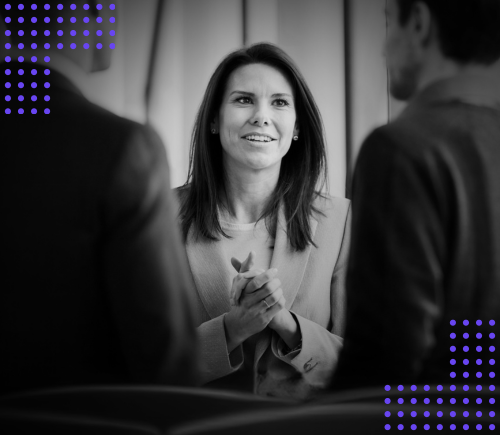
CISO New Zealand
18 Nov 2025
.png)
CISO Singapore
This event equips security leaders with critical strategies to combat the rapidly escalating cyber threats, while arming businesses with actionable solutions to align cyber security initiatives with business resilience, delivering ironclad defenses.
19-20 Aug 2025
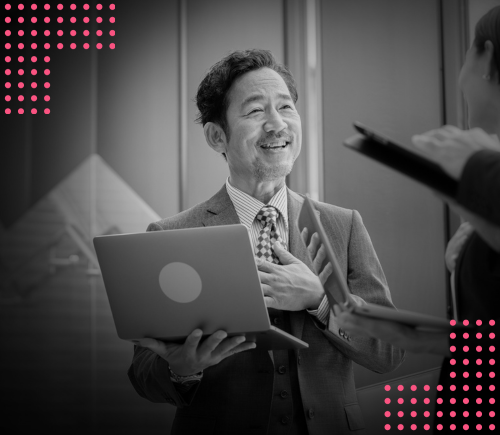
Cloud Security Singapore
Attend Cloud Security Singapore to find out how you can increase resilience through preparedness, mitigate AI risks, and implement cloud security governance for regulatory compliance, risk management, and data protection.
20 August 2025
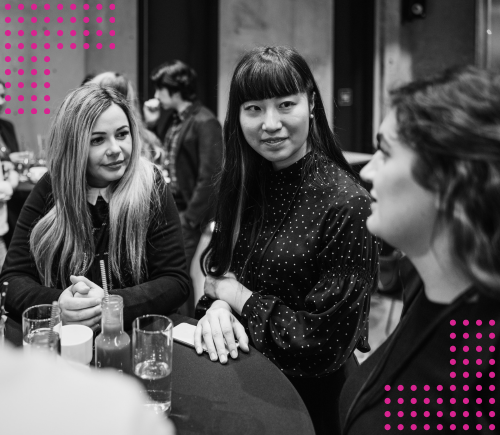
AppSec & DevSecOps Singapore
Join us and discover strategies to measure effectiveness, foster security culture, empower developers, integrate tools, and secure supply chains. Balance automation with human oversight for continuous improvement and risk mitigation.
20 August 2025
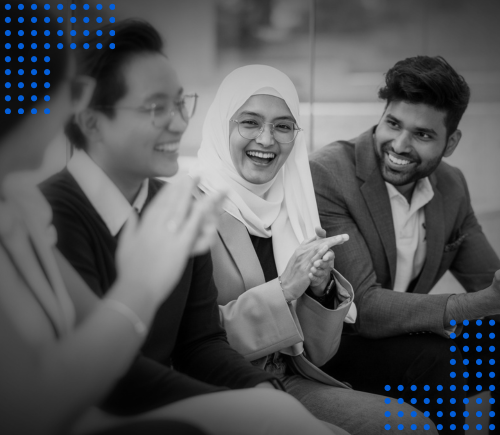
CDAO Indonesia
2026
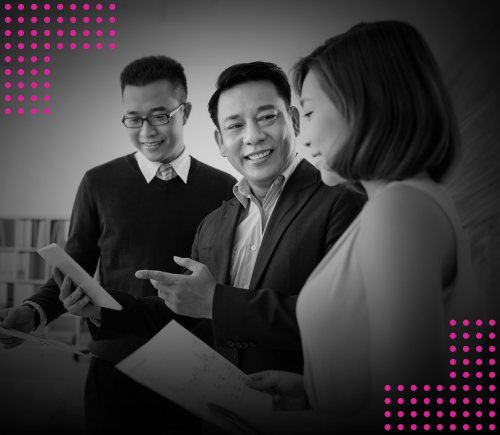
CISO FSI Singapore
22 Apr 2026 | Singapore
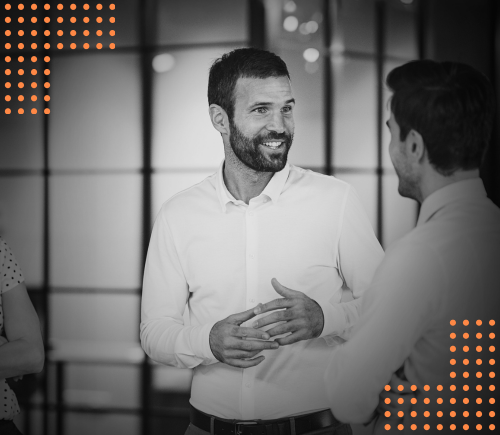
OT Security Melbourne 2025
Melbourne
22 July 2025
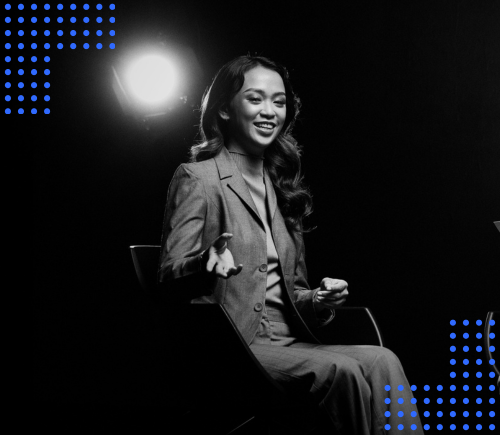
CDAO Philippines
Connecting you to what's next in data and AI
22 October 2025
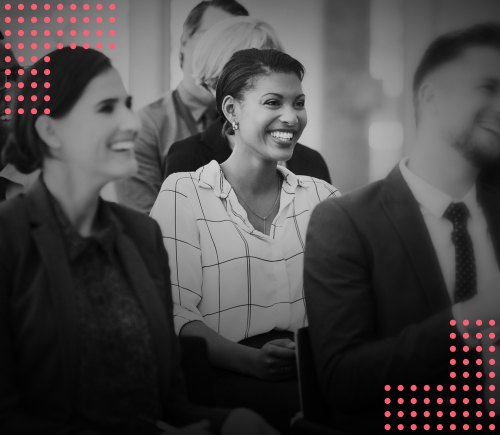
CISO Melbourne
22-23 July 2025
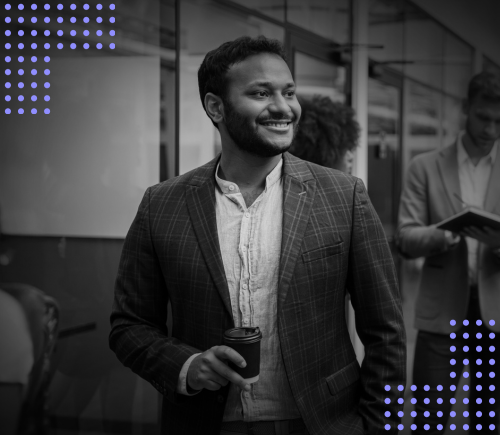
Cloud Security Melbourne
23 Jul 2025
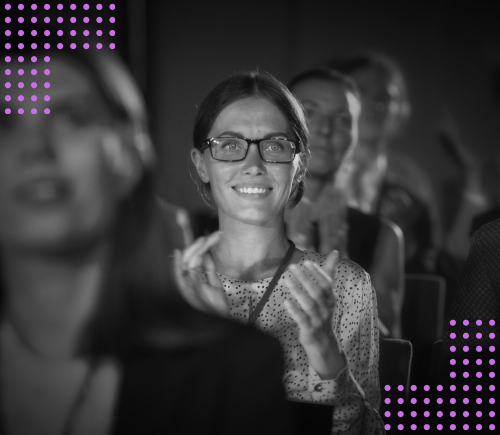
AppSec & DevSecOps Melbourne
23 Jul 2025 | Crown Promenade
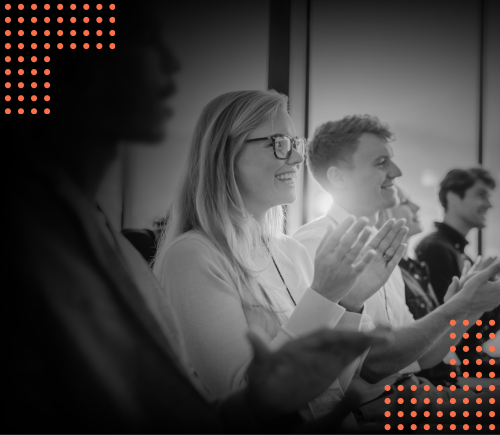
CISO Brisbane
Join prominent InfoSec leaders to get inspired, share intelligence and rekindle relationships
24 June 2025 | Brisbane
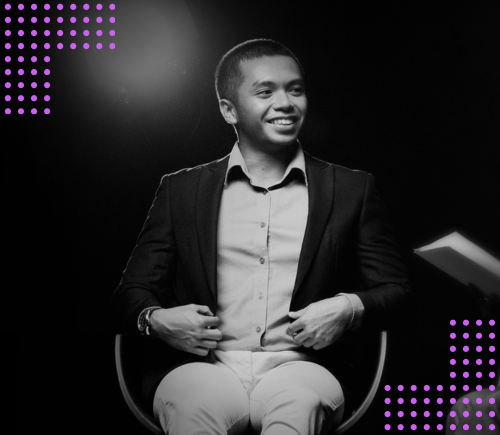
CISO Philippines
26 November 2025
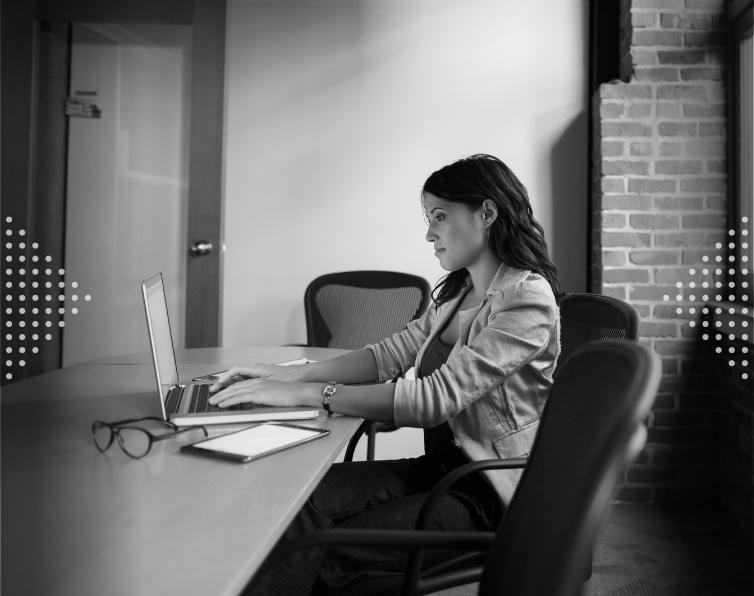
Critical Infrastructure ANZ Online
Online Event
28 March 2025
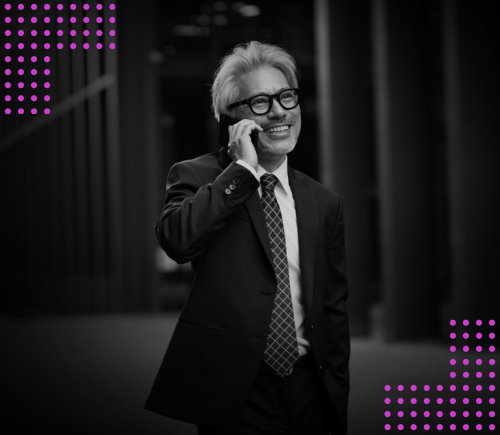
CISO Indonesia
28 May 2025
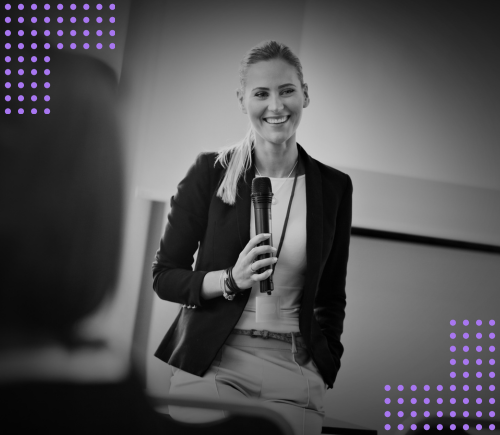
Modern DevOps Sydney
29 May 2024 | Sydney Central Hotel by The Ascott
-1.png)
CDAO Mexico
Empoderando a los Líderes para Impulsar la Innovación: Transformando los Datos y la Analítica en Tiempo Real
Ciudad de México
3 - 4 de junio, 2025
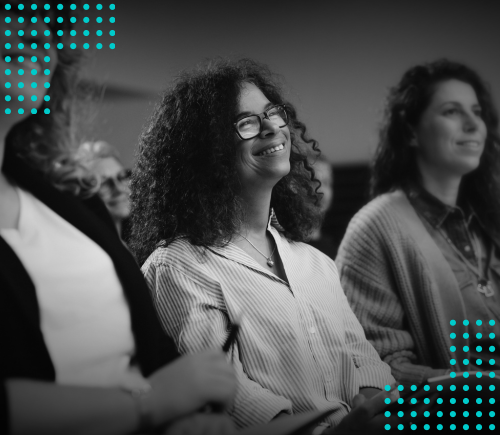
CDAO New Zealand
Chief Data & Analytics Officer (CDAO) New Zealand offers all organisations a senior-level platform to exchange data strategies, improve customer-centric delivery, and optimise intelligent decision-making, ensuring responsible use of AI and data analytics.
3-4 Nov 2025
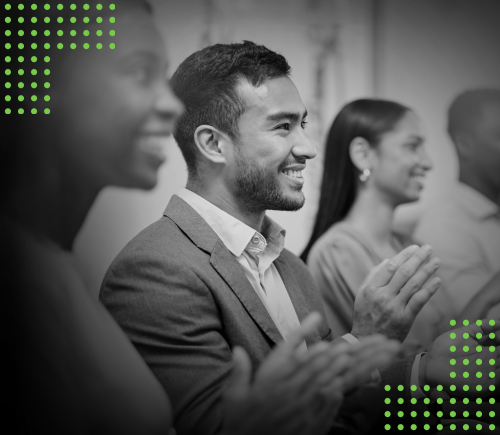
Data Architecture New Zealand
Data Architecture Auckland 2025 is your gateway to the future of data management and AI. Join us to elevate your expertise and redefine excellence.
4 Nov 2025 | Auckland
.png)
CDAO Brisbane
5 Mar 2026
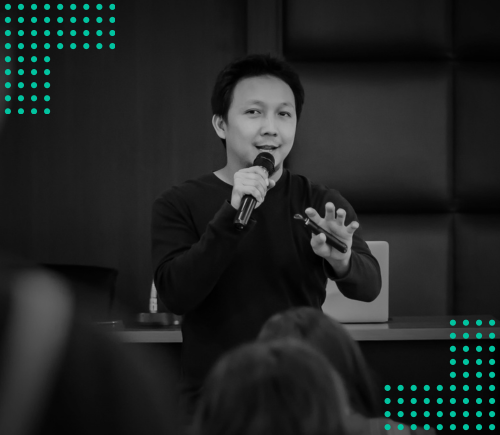
CDAO Malaysia
CDAO Malaysia is returning for its third year as the largest annual gathering of the nation’s most senior data, analytics, and AI decision-makers. Once again, data leaders are coming together to connect, learn and innovate towards a better future.
7 Oct 2025 | Kuala Lumpur
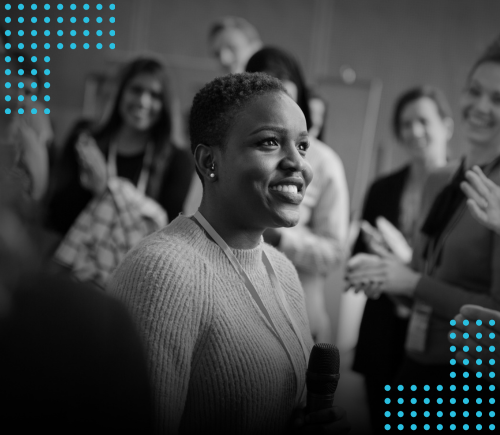
CDAO Sydney
Connecting you to what's next in data
7-8 May, 2026
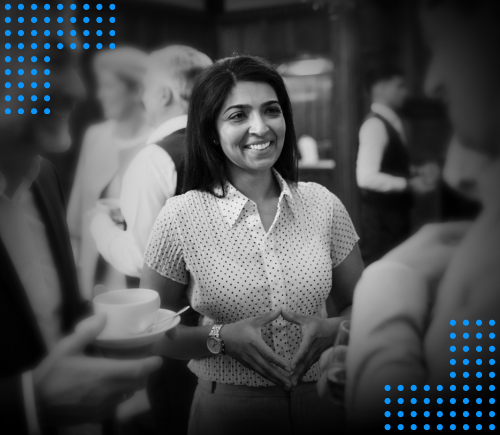
Data Architecture Sydney
8 May 2025 | Royal Randwick Racecourse
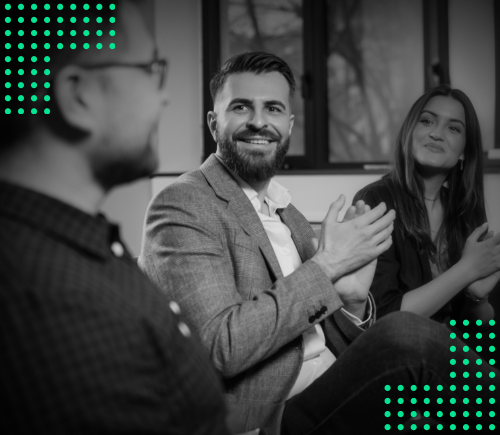
Enterprise AI Sydney
Accelerating digital transformation through AI innovation
8 May 2025 | Royal Randwick Racecourse
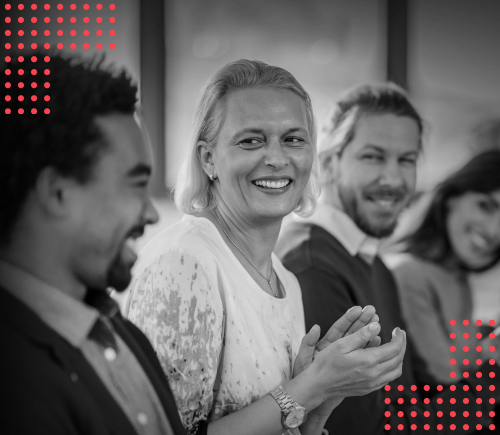
Modern DevOps Melbourne
8 October 2024 | Grand Hyatt Melbourne
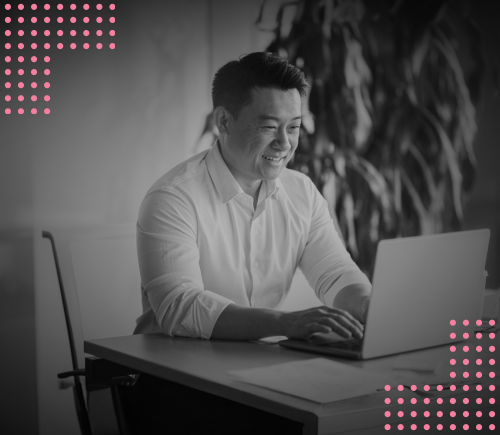
AI in Cyber ANZ Online
Virtual forum AI in Cyber provides essential tools to navigate the intersection of AI and security. Featuring keynotes and panel discussions, it covers everything from securing AI and ethical considerations to unlocking the full potential of AI.
9 Sept 2025
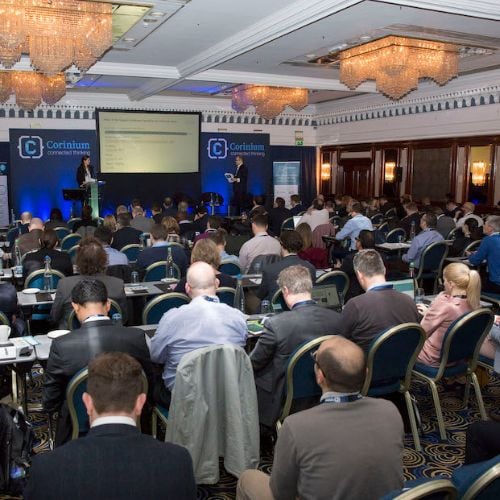
CDAO Financial Services & Insurance UK 2025
Europe's Most Senior Data & Analytics Event for Financial Services and Insurance
London
9 September 2025
.png)
CDAO Germany
Putting Data at the Heart of Business, Driving Value in Uncertain Times
Munich
April 16-17, 2024
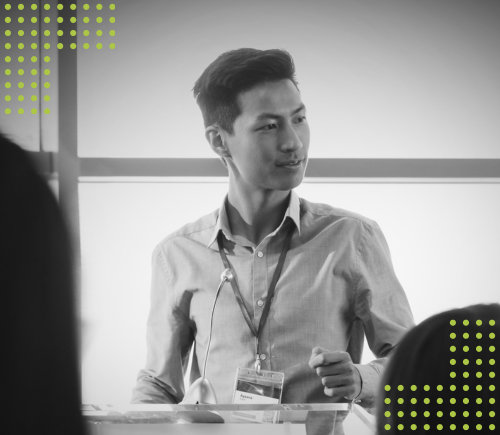
CDAO Singapore
The cross-industry exchange for all data and analytics leaders
April 2026
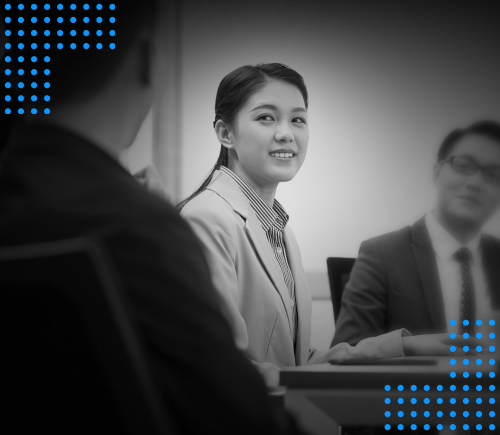
Data Architecture Singapore
April 2026
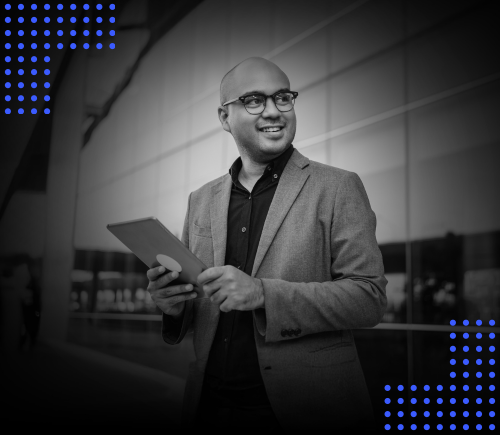
Enterprise AI Singapore
April 2026
.png)
CDAO Germany
Putting Data at the Heart of Business, Driving Value in Uncertain Times
Munich
April 28-29, 2026
.png)
CDAO Germany
Putting Data at the Heart of Business, Driving Value in Uncertain Times
Munich
April 29-30, 2025
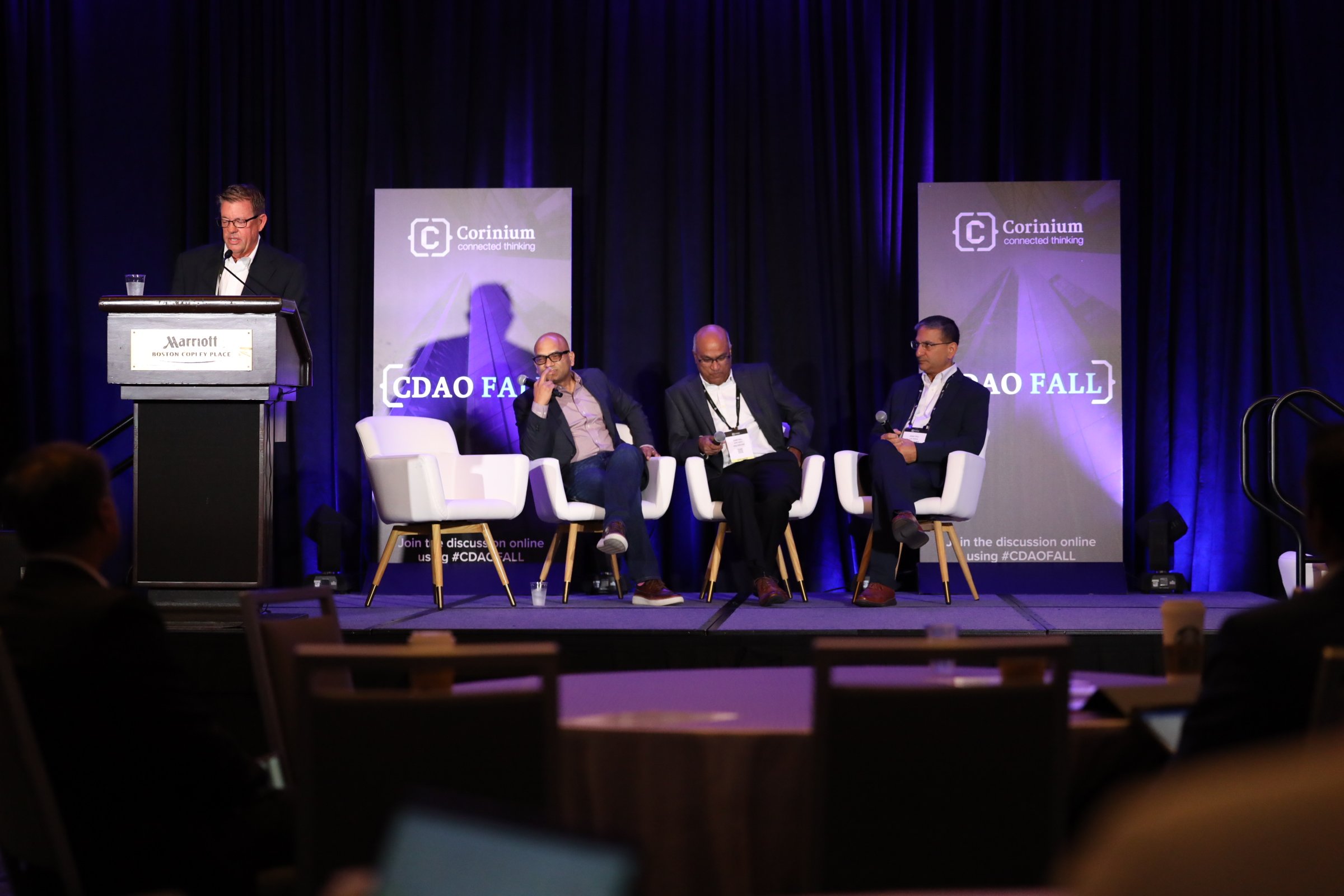
CDAO Chicago
Reconnecting Chicago's Data & Analytics Leaders In-Person to Accelerate Their Data Transformation Strategies
Chicago
August 13-14, 2025
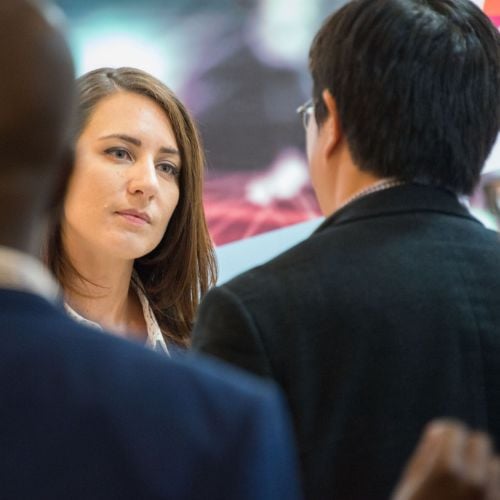
CDAO Fed Ready Summit
Shape the Future of Federal Data & AI: Join 50 Top Leaders Preparing for 2025
Washington, DC Metro Area (Arlington, VA.)
December 11, 2025 | Convene 1201 Wilson Blvd
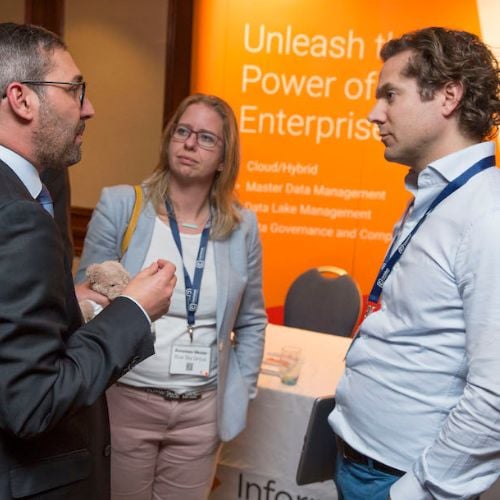
CDAO Dallas 2025
Join your data & analytics peers from Dallas as you discover the latest trends and challenges facing your role
Dallas
December 2, 2025
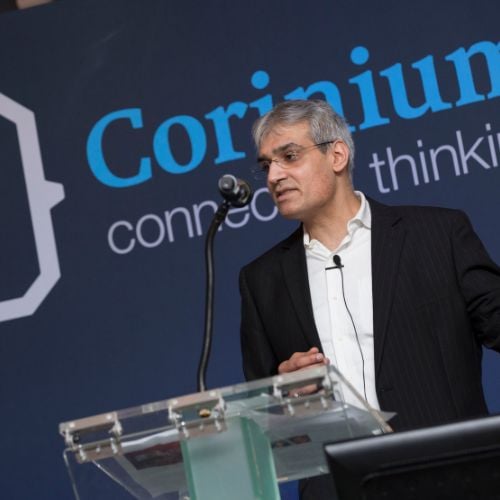
CDAO Financial Services
Focused on data-driven strategies, this conference covers topics like AI, risk management, regulatory compliance, and enhancing customer experience. Through interactive sessions, workshops, and networking opportunities, attendees will gain actionable insights to optimize their data and analytics efforts, driving innovation and growth within their organizations.
New York, NY
February 18-19, 2026
.png)
CDAO Insurance
With a focus on data-driven innovation, this conference covers critical areas like AI applications in underwriting, risk analytics, compliance, and enhancing the policyholder experience. Through expert-led sessions, practical workshops, and high-impact networking opportunities, attendees will gain actionable strategies to optimize data and analytics within their organizations, fostering resilience, efficiency, and growth.
New York, NY
February 19, 2026
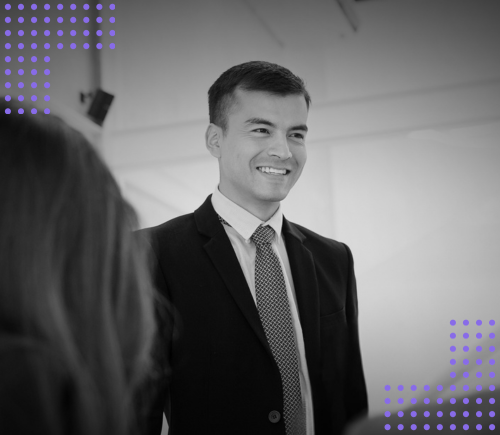
CISO Malaysia
February 2026
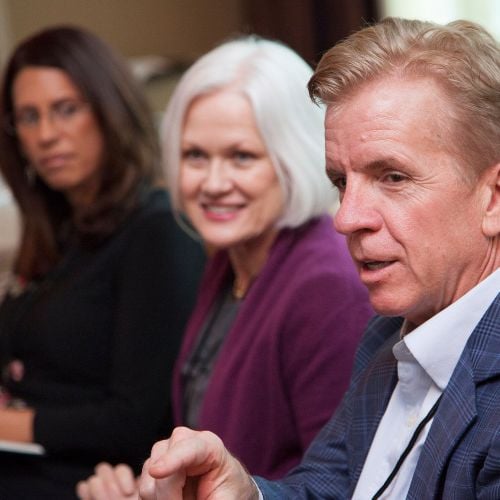
CDAO UK 2026
Join the UK's most senior data & analytics event
London
February 25-26, 2026
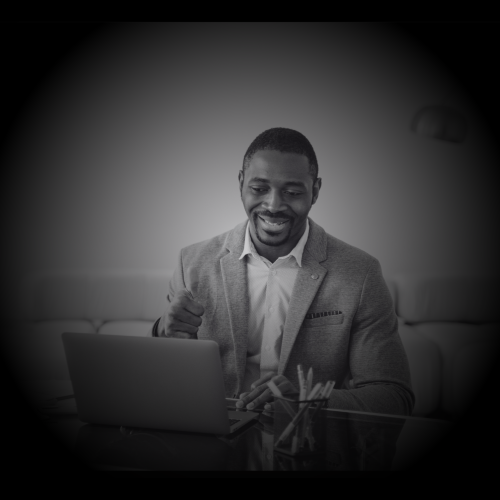
Location Intelligence in Financial Services Online
Discover how geospatial data and AI-driven analytics are transforming financial services. From customer engagement to fraud detection and risk management, explore real-world applications, regulatory insights, and the future of location intelligence in banking and insurance.
Online
July 16, 2025
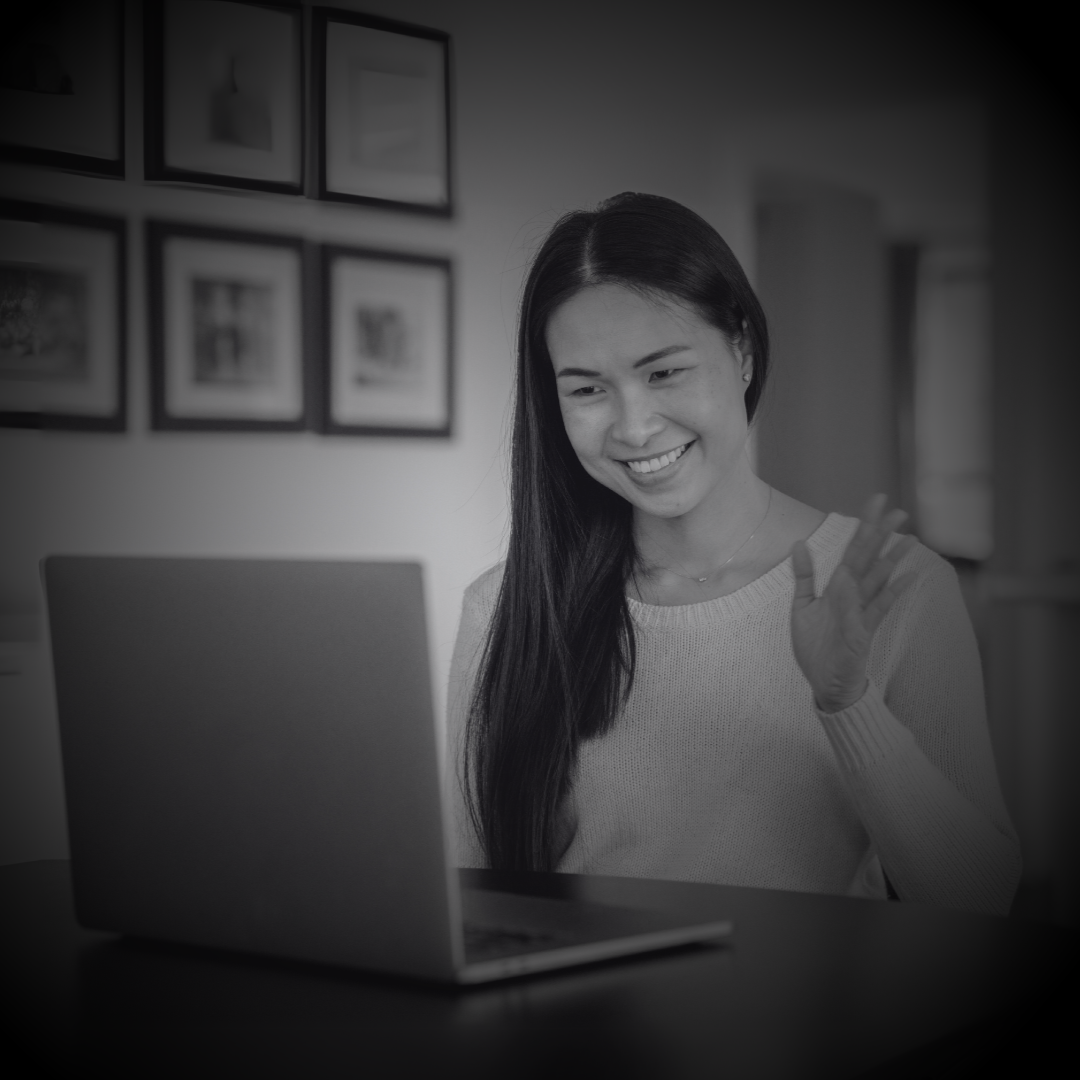
Data & Analytics Live
Leading Virtual Event for Data & Analytics Executives in the Age of Artificial Intelligence
Online Event
July 9, 2025
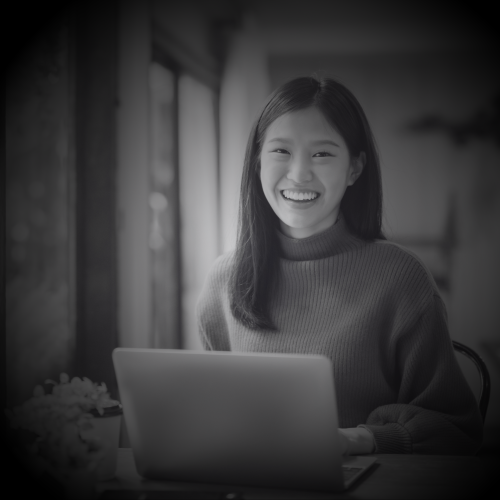
AI Risk & Compliance Online
Explore how artificial intelligence is transforming risk management, regulatory compliance, and fraud detection. Industry experts will discuss AI-driven strategies, emerging trends, and best practices to enhance security, accuracy, and efficiency in compliance processes.
Online
June 18th, 2025
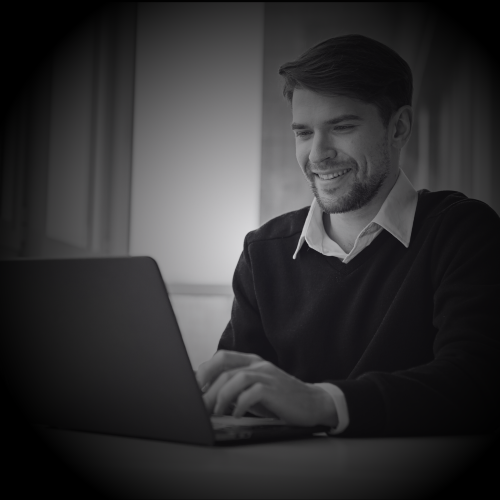
Agentic AI in Financial Services Online
Discover the impact of autonomous AI systems in financial services. Experts will discuss how agentic AI is revolutionizing trading, risk management, fraud detection, and customer interactions. Learn about cutting-edge innovations, ethical considerations, and real-world applications driving the future of finance.
Online
June 25, 2025
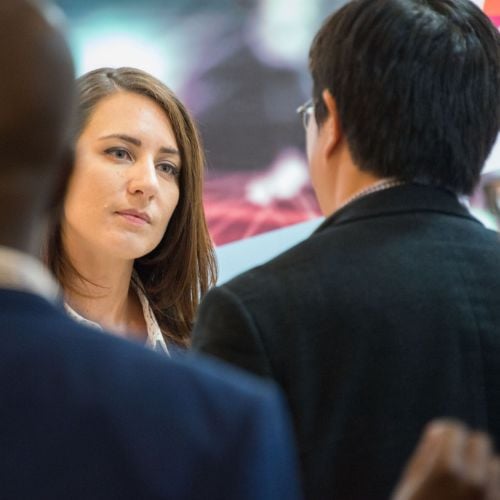
CDAO Government
The Longest Running Event for Federal, State, and Local Government Data & Analytics Leaders
Washington, DC Metro Area (Arlington, VA.)
June 25-26, 2025 | Convene 1201 Wilson Blvd
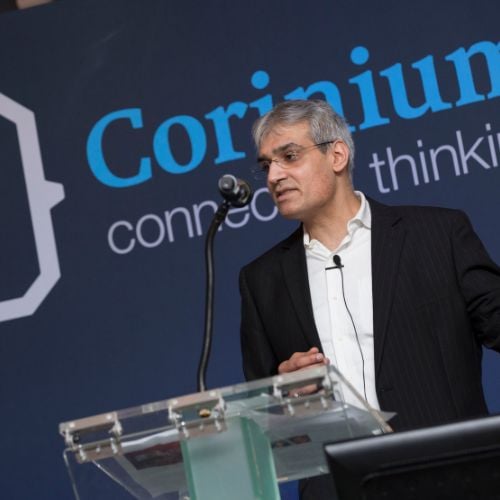
CDAO Spain
Únete al evento referente en Datos y Analítica de España
Madrid
Junio, 2026
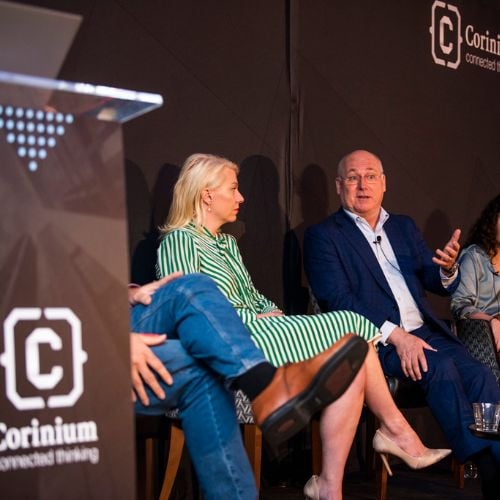
CDAO France
Conçu pour les leaders des données stratégiques et de l'analyse des plus grandes marques françaises, CDAO France sera le mélange parfait d'informations pratiques et de réseautage de haut niveau.
Paris
Mai 21 2026
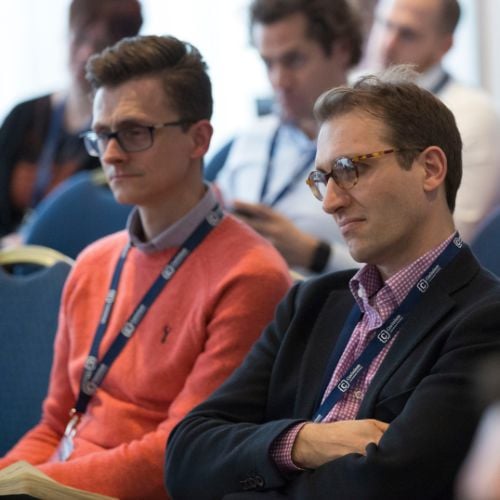
CDAO Canada
Leveraging Generative AI to Transform Your Data and Analytics Strategy to Deliver Enhanced Business Innovation
Toronto
March, 2026
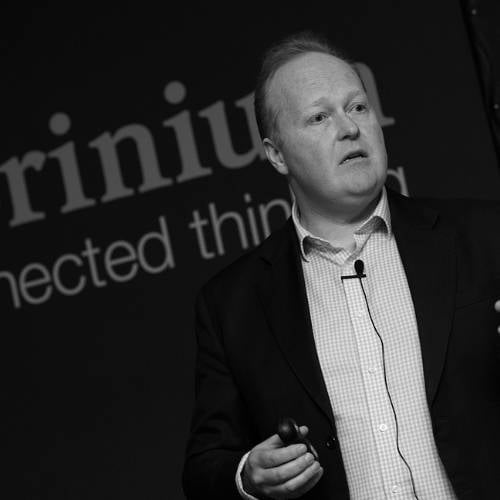
CISO Chicago
Top executives and industry leaders come together to tackle the latest challenges in cybersecurity.
Chicago
March, 2026
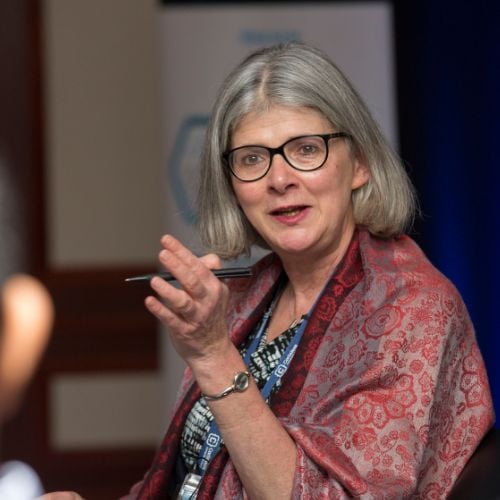
CDAO West Coast
Explore the latest innovations, strategies, and trends driving the future of data and analytics. This exclusive event brings together leaders from various industries to engage in insightful discussions, hands-on workshops, and high-level networking opportunities.
San Jose
May 13, 2026
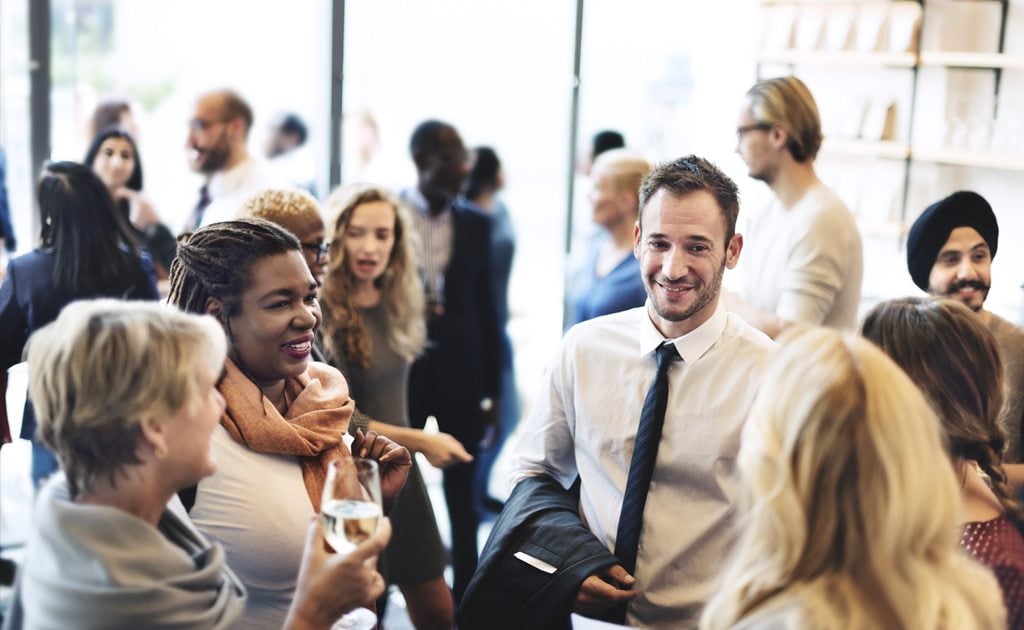
CISO Public Sector Online A/NZ
Reinforcing public sector cyber security resilience in Australia and New Zealand
Online
May 2, 2023
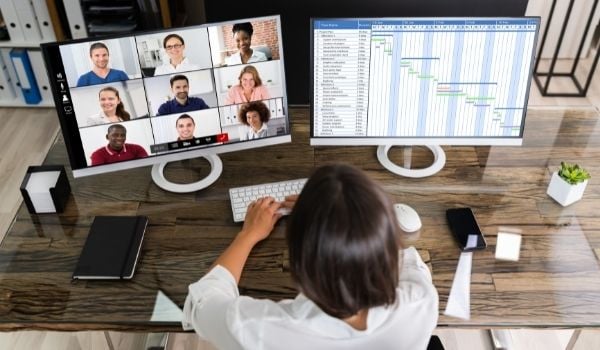
CISO ASEAN Online
Online
May 30, 2023
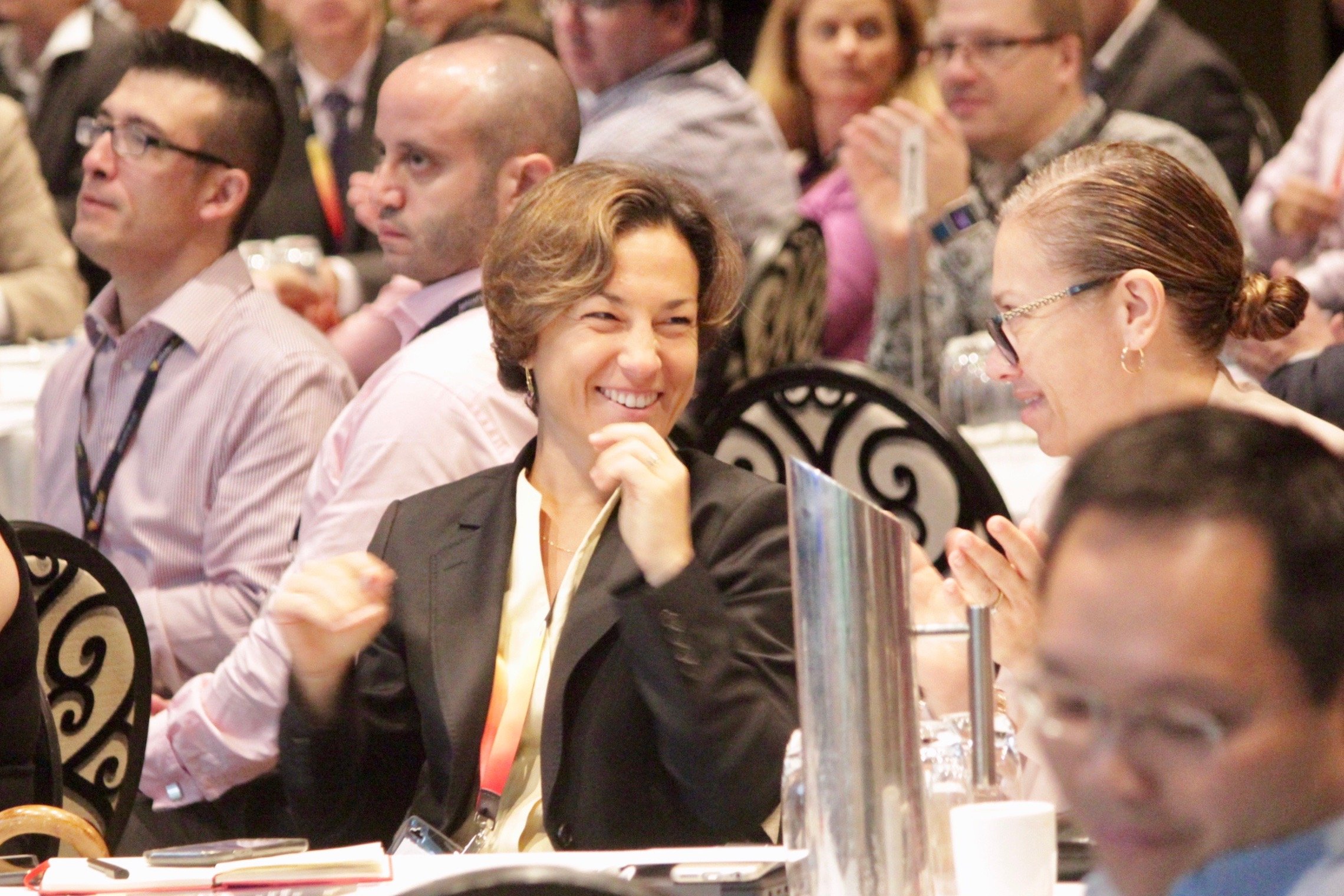
CDAO Europe 2024
Europe's Foremost Data Event for Top Leaders Across The Benelux And Beyond
Amsterdam
November 12, 2024
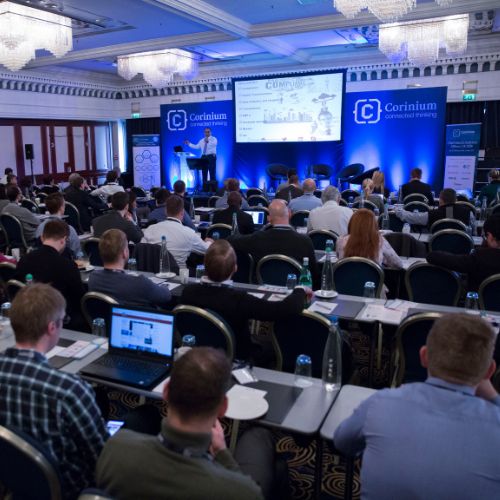
Future-Proof Your Marketing: How Secret Escapes Built a First-Party Data Strategy
Join us for an insightful webinar on revolutionizing Digital Analytics strategies to meet the new industry standard on first-party cookies. Explore how ‘Next-Gen Digital Analytics’ is meticulously crafted to assist our customers in modernizing their data strategy and future-proofing their marketing stack. Powered by core AWS and Snowflake components. Don’t miss out on this opportunity to elevate your Digital Analytics strategy to the next level!
Virtual Webinar
November 12, 2024
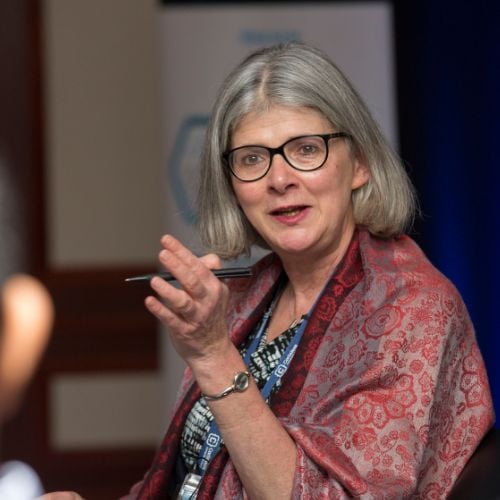
CDAO Benelux 2025
Europe's Foremost Data Event for Top Leaders Across The Benelux And Beyond
Amsterdam
November 12, 2025
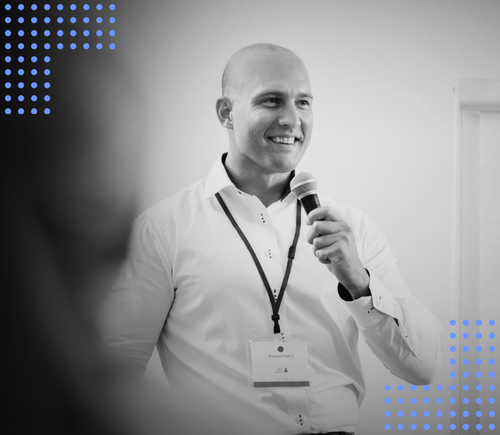
Autonomy in Defense
Accelerating Mission Success Through Trusted Autonomous Systems
Washington D.C.
November 12-13, 2025
.png)
CDAO Nordics
Practical insights for data leaders
Stockholm
November 19, 2024
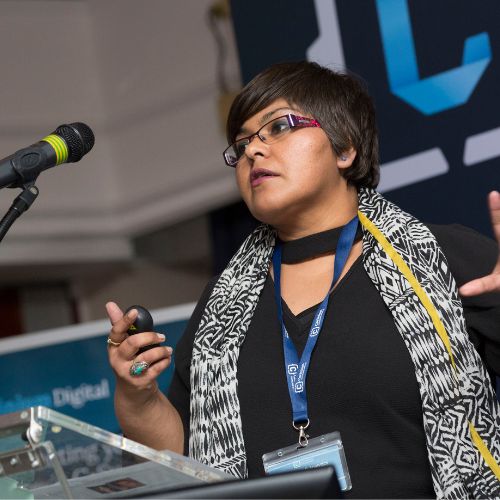
CDAO Nordics 2025
The leading event in Nordics delivering practical insights for data and analytics leaders
Stockholm
November 5, 2025
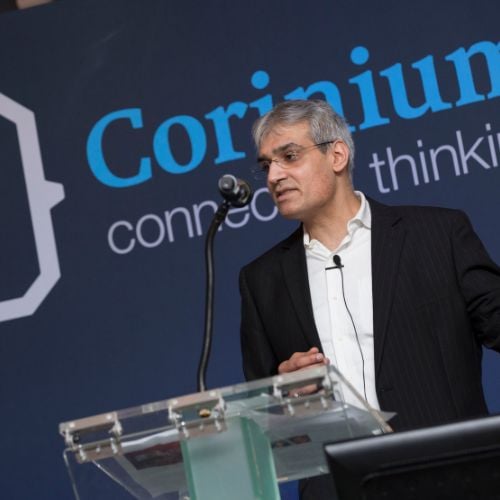
CDAO Fall
Ignite the Data Revolution – Join the Elite Vanguard of Leaders Shaping the Future with Game-Changing AI, Advanced Analytics, and Data-Driven Transformation Strategies
Boston
October 22-23, 2025
.png)
CDAO Frankfurt
Putting Data at the Heart of Business, Driving Value in Uncertain Times
Frankfurt
October 30, 2024
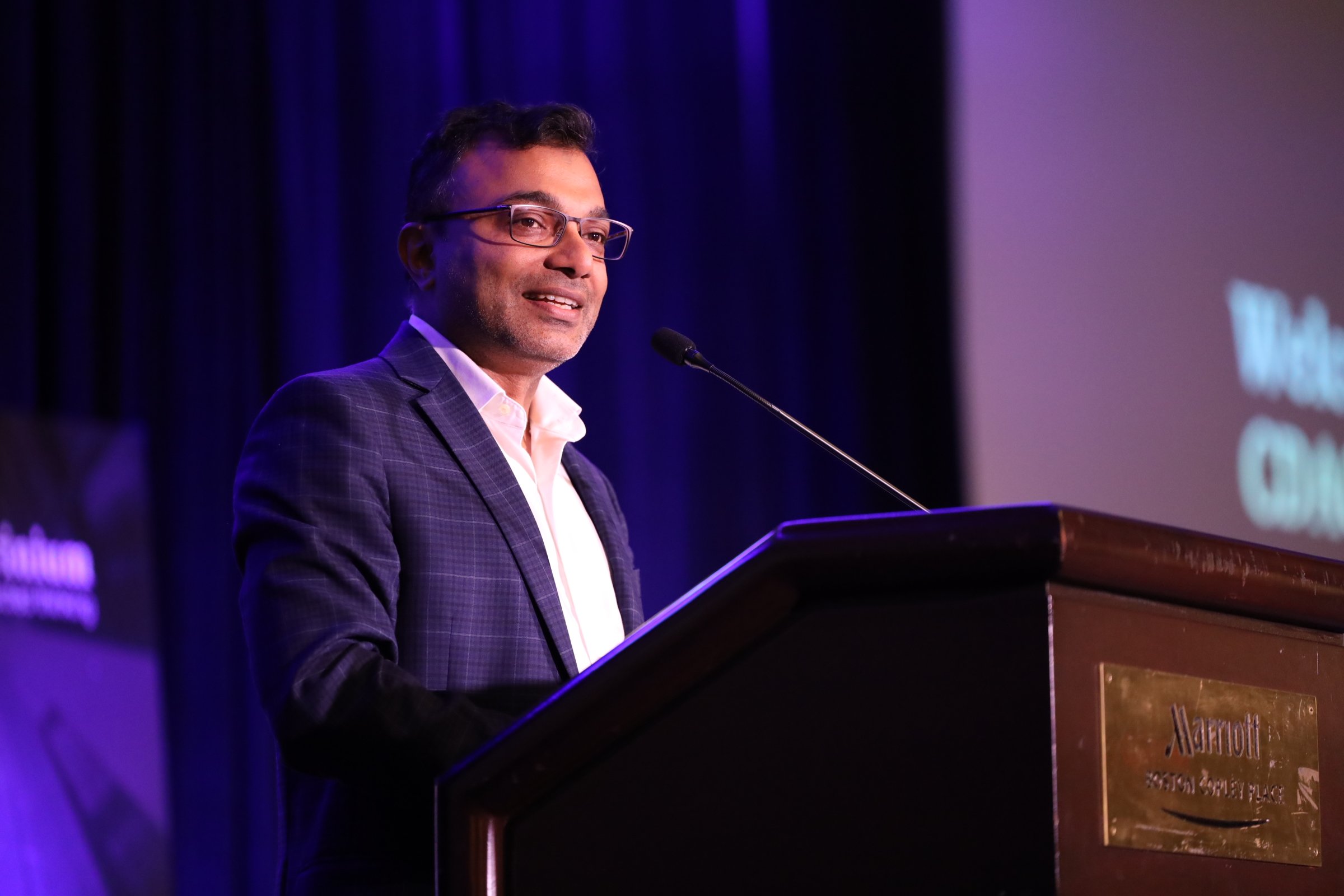
CDAO Canada Public Sector
The Premier Event for Canada's Public Sector Data & Analytics Leaders
Ottawa
October 8-9, 2025
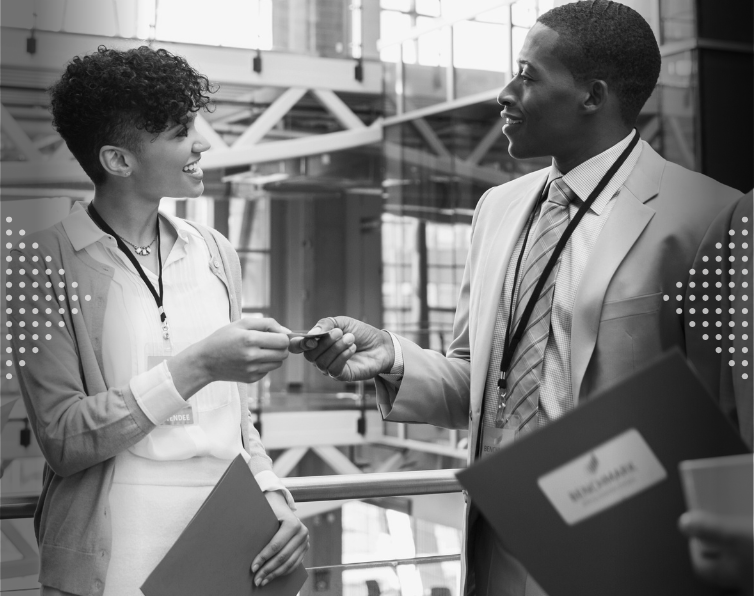
CDAO Defense & Security 2025
Explore the critical role of data-driven decision-making, AI applications, and emerging technologies in enhancing national security, operational effectiveness, and mission success.
Washington D.C.
September 16-17, 2025
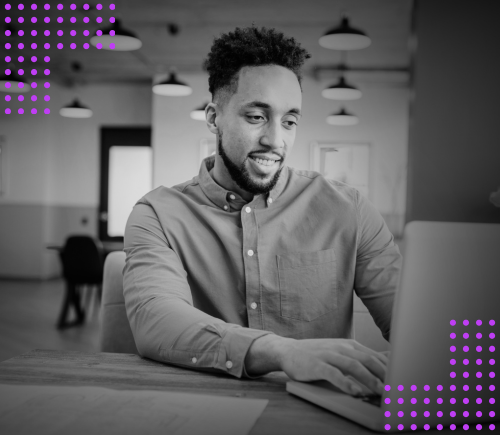
CDAO Government Online
CDAO Government Online is the leading virtual event empowering public sector leaders to harness data and AI for smarter, more effective government.
Online
September 23, 2025 | Virtual
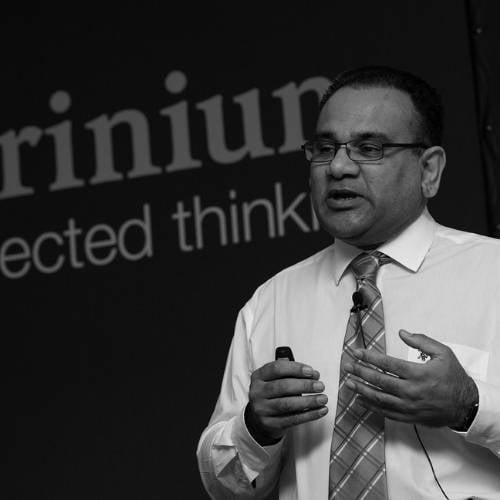
CISO NY
Top executives and industry leaders come together to tackle the latest challenges in cybersecurity.
New York
September 9, 2025